Radiomic Features on Prostatic Multiparametric Magnetic Resonance Imaging Enable Progression Risk in Patients on Active Surveillance: A Pilot Study
Article Information
Angelo Totaro1*, Valerio Di Paola2, Marco Campetella1, Eros Scarciglia1, Luca Boldrini3, Riccardo Manfredi2, Pierfrancesco Bassi1, Lorenzo Elia2, Riccardo Gigli2, Federica Perillo2, Davide Cusumano4, Antonio Cretì1, Sebastiano Vocino1, Luigi Cosenza1, Francesco Pio Bizzarri1, Filippo Marino1, Francesco Pinto1, Emilio Sacco1
Affiliation:
1Catholic University of the Sacred Heart, Fondazione Policlinico 'A. Gemelli', Urological Clinic, Rome, Italy.
2Department of Radiology, Catholic University of the Sacred Heart, Fondazione Policlinico "A. Gemelli", 00168 Rome, Italy.
3Department of Radiotherapy, Catholic University of the Sacred Heart, Fondazione Policlinico "A. Gemelli", 00168 Rome, Italy.
4Department of Medical Physics, Catholic University of the Sacred Heart, Fondazione Policlinico "A. Gemelli", 00168 Rome, Italy.
*Corresponding author: Angelo Totaro, Catholic University of the Sacred Heart, Fondazione Policlinico 'A. Gemelli', Urological Clinic, Rome, Italy.
Received: November 27, 2022; Accepted: December 05, 2022; Published: December 15, 2022
Citation:
Angelo Totaro, Valerio Di Paola, Marco Campetella, Eros Scarciglia, Luca Boldrini, Riccardo Manfredi, Pierfrancesco Bassi, Lorenzo Elia, Riccardo Gigli, Federica Perillo, Davide Cusamano, Antonio Cretì, Sebastiano Vocino, Luigi Cosenza, Francesco Pio Bizzarri, Filippo Marino, Francesco Pinto, Emilio Sacco. Radiomic Features on Prostatic Multiparametric Magnetic Resonance Imaging Enable Progression Risk In Patients on Active Surveillance: A Pilot Study. Journal of Radiology and Clinical Imaging 5 (2022): 78-86.
Share at FacebookAbstract
Purpose: Active Surveillance (AS) for not clinically significant prostate cancer (ncs-PCa) has increased worldwide in the last 10 years. However, about 30-40% patients experience progression to clinically significant prostate cancer (csPCa). To date, there is no validated protocol for followup of AS patients. Hence quantitative analysis for imaging data using radiomics has become an emerging paradigm. Quantitative radiomics allows for the extraction of more advanced shape features. Our aim was to assess which shape features derived from MRI index lesions correlate with risk progression to csPCa, in patients on AS.
Materials and Methods: We retrospectively enrolled 55 patients’ ncs-PCa, according to Epstein criteria. All patients underwent internal AS protocol: PSA every 3 months; MRI at 9 months and then yearly; confirmatory biopsy at 12 months. Diagnosis of ISUP GG>2 or highvolume ISUP GG1 disease (defined as increase >20% volume disease from baseline biopsy) on confirmatory biopsy were considered as progression to csPCa. All MRIs were evaluated and manually contoured by 2 expert radiologists at our institution. Statistical analysis evaluated 270 radiomic features, extracted from lesion index after tridimensional segmentation. Predictive models were created with the best performing radiomic features and clinical variables, considering the area under the Receiver Operating Characteristic (ROC) curve (AUC).
Results: Progression rate to csPCa was 40% (22/55), at median follow up of 2 years. Univariate analysis showed 10 radiomic features that were found to be significantly associated to progression to csPCa (5 extracted from DWI and 5 from T2w MR maps). The predictive model with the two best performing radiomic features showed an AUC of 0.76 (95% CI of 0.62-0.89) in predicting progression to csPCa, with a sensitivity of 76.2% and a specificity of 66.7%. On multivariate analysis, age
Keywords
Rdiomic; Active surveillance; DWI maps; Predictive model
Rdiomic articles; Active surveillance articles; DWI maps articles; Predictive model articles
Radiomic articles Radiomic Research articles Radiomic review articles Radiomic PubMed articles Radiomic PubMed Central articles Radiomic 2023 articles Radiomic 2024 articles Radiomic Scopus articles Radiomic impact factor journals Radiomic Scopus journals Radiomic PubMed journals Radiomic medical journals Radiomic free journals Radiomic best journals Radiomic top journals Radiomic free medical journals Radiomic famous journals Radiomic Google Scholar indexed journals Active surveillance articles Active surveillance Research articles Active surveillance review articles Active surveillance PubMed articles Active surveillance PubMed Central articles Active surveillance 2023 articles Active surveillance 2024 articles Active surveillance Scopus articles Active surveillance impact factor journals Active surveillance Scopus journals Active surveillance PubMed journals Active surveillance medical journals Active surveillance free journals Active surveillance best journals Active surveillance top journals Active surveillance free medical journals Active surveillance famous journals Active surveillance Google Scholar indexed journals DWI maps articles DWI maps Research articles DWI maps review articles DWI maps PubMed articles DWI maps PubMed Central articles DWI maps 2023 articles DWI maps 2024 articles DWI maps Scopus articles DWI maps impact factor journals DWI maps Scopus journals DWI maps PubMed journals DWI maps medical journals DWI maps free journals DWI maps best journals DWI maps top journals DWI maps free medical journals DWI maps famous journals DWI maps Google Scholar indexed journals Predictive model articles Predictive model Research articles Predictive model review articles Predictive model PubMed articles Predictive model PubMed Central articles Predictive model 2023 articles Predictive model 2024 articles Predictive model Scopus articles Predictive model impact factor journals Predictive model Scopus journals Predictive model PubMed journals Predictive model medical journals Predictive model free journals Predictive model best journals Predictive model top journals Predictive model free medical journals Predictive model famous journals Predictive model Google Scholar indexed journals not clinically significant prostate cancer articles not clinically significant prostate cancer Research articles not clinically significant prostate cancer review articles not clinically significant prostate cancer PubMed articles not clinically significant prostate cancer PubMed Central articles not clinically significant prostate cancer 2023 articles not clinically significant prostate cancer 2024 articles not clinically significant prostate cancer Scopus articles not clinically significant prostate cancer impact factor journals not clinically significant prostate cancer Scopus journals not clinically significant prostate cancer PubMed journals not clinically significant prostate cancer medical journals not clinically significant prostate cancer free journals not clinically significant prostate cancer best journals not clinically significant prostate cancer top journals not clinically significant prostate cancer free medical journals not clinically significant prostate cancer famous journals not clinically significant prostate cancer Google Scholar indexed journals clinically significant prostate cancer articles clinically significant prostate cancer Research articles clinically significant prostate cancer review articles clinically significant prostate cancer PubMed articles clinically significant prostate cancer PubMed Central articles clinically significant prostate cancer 2023 articles clinically significant prostate cancer 2024 articles clinically significant prostate cancer Scopus articles clinically significant prostate cancer impact factor journals clinically significant prostate cancer Scopus journals clinically significant prostate cancer PubMed journals clinically significant prostate cancer medical journals clinically significant prostate cancer free journals clinically significant prostate cancer best journals clinically significant prostate cancer top journals clinically significant prostate cancer free medical journals clinically significant prostate cancer famous journals clinically significant prostate cancer Google Scholar indexed journals area under the Receiver Operating Characteristic articles area under the Receiver Operating Characteristic Research articles area under the Receiver Operating Characteristic review articles area under the Receiver Operating Characteristic PubMed articles area under the Receiver Operating Characteristic PubMed Central articles area under the Receiver Operating Characteristic 2023 articles area under the Receiver Operating Characteristic 2024 articles area under the Receiver Operating Characteristic Scopus articles area under the Receiver Operating Characteristic impact factor journals area under the Receiver Operating Characteristic Scopus journals area under the Receiver Operating Characteristic PubMed journals area under the Receiver Operating Characteristic medical journals area under the Receiver Operating Characteristic free journals area under the Receiver Operating Characteristic best journals area under the Receiver Operating Characteristic top journals area under the Receiver Operating Characteristic free medical journals area under the Receiver Operating Characteristic famous journals area under the Receiver Operating Characteristic Google Scholar indexed journals Multiparametric Magnetic Resonance Imaging of the Prostate articles Multiparametric Magnetic Resonance Imaging of the Prostate Research articles Multiparametric Magnetic Resonance Imaging of the Prostate review articles Multiparametric Magnetic Resonance Imaging of the Prostate PubMed articles Multiparametric Magnetic Resonance Imaging of the Prostate PubMed Central articles Multiparametric Magnetic Resonance Imaging of the Prostate 2023 articles Multiparametric Magnetic Resonance Imaging of the Prostate 2024 articles Multiparametric Magnetic Resonance Imaging of the Prostate Scopus articles Multiparametric Magnetic Resonance Imaging of the Prostate impact factor journals Multiparametric Magnetic Resonance Imaging of the Prostate Scopus journals Multiparametric Magnetic Resonance Imaging of the Prostate PubMed journals Multiparametric Magnetic Resonance Imaging of the Prostate medical journals Multiparametric Magnetic Resonance Imaging of the Prostate free journals Multiparametric Magnetic Resonance Imaging of the Prostate best journals Multiparametric Magnetic Resonance Imaging of the Prostate top journals Multiparametric Magnetic Resonance Imaging of the Prostate free medical journals Multiparametric Magnetic Resonance Imaging of the Prostate famous journals Multiparametric Magnetic Resonance Imaging of the Prostate Google Scholar indexed journals Radiology articles Radiology Research articles Radiology review articles Radiology PubMed articles Radiology PubMed Central articles Radiology 2023 articles Radiology 2024 articles Radiology Scopus articles Radiology impact factor journals Radiology Scopus journals Radiology PubMed journals Radiology medical journals Radiology free journals Radiology best journals Radiology top journals Radiology free medical journals Radiology famous journals Radiology Google Scholar indexed journals Treatment Planning System articles Treatment Planning System Research articles Treatment Planning System review articles Treatment Planning System PubMed articles Treatment Planning System PubMed Central articles Treatment Planning System 2023 articles Treatment Planning System 2024 articles Treatment Planning System Scopus articles Treatment Planning System impact factor journals Treatment Planning System Scopus journals Treatment Planning System PubMed journals Treatment Planning System medical journals Treatment Planning System free journals Treatment Planning System best journals Treatment Planning System top journals Treatment Planning System free medical journals Treatment Planning System famous journals Treatment Planning System Google Scholar indexed journals
Article Details
Prostate cancer (PCa) is the second most common malignancy diagnosed in men, and the fifth deadliest worldwide [1]. Nearly half of men present at diagnosis with low or favourable intermediate risk PCa and may be considered suitable for Active Surveillance (AS) [2,3]. This kind of treatment, alternative to the surgery, has increased worldwide in the last 10 years, as only a small part of patients experiences tumor progression to clinically significant prostate cancer (csPCa) [4]. Many attempts have been made in order to increase the number of AS-suitable patients, without increasing disease misclassification risk. However, standardized protocols for the management of patients in active surveillance are still lacking. The lack of consensus on both the stringency of inclusion criteria and the definition of disease progression has led to a significant variability of AS protocols across centers. AS requires a closely monitoring of patient with periodic Multiparametric Magnetic Resonance Imaging of the Prostate (mpMRI) and Prostate Biopsies (PBx). There is no consensus on the right timing of mpMRI and prostate biopsy. As a result, a cumulative five-year dropout rate on AS reaches 44%, of which 27% are triggered by disease progression [5]. A lot of nomograms and individual clinicopathological predictors of histopathological progression in AS cohorts has been investigated in the last years, as summarized in a systematic review by Sierra et al. [6], but none of the proposed models has entered in routine clinical practice because of low predictive accuracy and poor performance with external validation. Actually, the most used tools in AS protocols are the follow: total PSA dosage, recurring mpMRI of the prostate and PBx. Moreover, mpMRI plays a key role in this setting, allowing to visualize the progression of the target lesion as well as the increase in the whole tumour. Despite the introduction of the second version of Prostate Imaging-Reporting and Data System (PI-RADS v2.1) to simplify terminology and mpMRI report content, subjectivity and interobserver variability is still present [7]. However, MRI provides the potential to investigate the ability of quantitative image-derived features through radiomic approaches, with the aim to develop accurate and reproducible predictors of disease progression. Radiomics has the great potential of extracting from the digital images quantitative information difficult to be revealed by the human eye, like the enhancement of edges and the variation of the grey level intensities within the region of interest, and translating these radiomic features into promising tools that can enhance diagnostic performance and guide the clinicians in the management of these patients [8,9]. In a recent study, Wang et al. demonstrated that a machine-learning classifier based on radiomics can improve the predictive performance of PI-RADS v2.1 score system [10]. Zhang et al. [11] recently introduced a radiomic model for predicting histopathological upgrading of PCa from biopsy to radical prostatectomy. Furthermore, radiomics models have been developed to preoperatively predict the probability of extracapsular extension [12,13]. To the best of our knowledge, only few studies reported the potential role of radiomics in predicting prostate cancer progression in patients on active surveillance [14,15]. In this retrospective study, we sought to develop a radiomics model able to predict the baseline risk of PCa progression in patients suitable for AS.
Materials And Methods
Patient population
This retrospective case-control study was part of experimental protocol on prostate cancer diagnosis, approved by local Institutional Review Board, and written informed consents were obtained from all patients before prostate biopsy.
We included all men with diagnosis of low risk PCa, according to the expanded Epstein criteria: PSA less than 10 ng/ml, clinical stage T1c-T2a, biopsy Gleason Score 6, maximum 4 cores involved, core invasion less than 50% [16].
Exclusion criteria were the follow: presence of other cancer; history of prostate surgery or pelvic surgery/ radiotherapy; prostatitis in the last six months; assumption of the phosphodiesterase 5-inhibitors and/or any androgen deprivation therapy in the last 6 months. Figure 1 depicts the scheme of internal AS protocol. Patients were divided into

Figure 1: Flowchart of AS protocol applied at our institution. AS= Active Surveillance; mpMRI= Multiparametric Magnetic Resonance of the Prostate; PCa= Prostate Cancer; PSA= Prostate Specific Antigen; RP= Radical Prostatectomy; SB= Standard Biopsy; TB= Targeted Biopsy.
two groups according to the disease progression status. The case group was patients who have progressed to csPCa, which was defined as histopathological progression on biopsy. The control group included patients without disease progression over the same follow-up period.
Targeted biopsy protocol
All biopsies were performed by expert urologists. Depending on clinical recommendations, patients with any suspicious for prostate cancer underwent a standard 12-core trans-perineal TRUS-guided extended Sextant Biopsy (SB) and MRI-targeted fusion biopsies (MRI-TBx,
≥3 cores/target) of mpMRI target lesions, performed by the same operator by using an office-based fusion platform (Esaote MyLabTwice® Navisuite 5.x navigation system, South Korean). We considered as suspicious all the PI- RADS≥3 MRI lesions. Prebiopsy T2-weighted MR images were segmented, registered, and fused with the real-time TRUS images. Tissue samples were sent for histopathologic evaluation by two expert pathologists, and biopsy results were used as the standard for assessment of the presence of cancer and disease progression.
Predictors of disease progression
To evaluate the role of clinicopathological predictors of disease progression, we collected the following parameters: age, total e free PSA, PSA density, MRI total prostate volume, PIRADS v2.1 score, target lesion size; ISUP-grade group and target lesion biopsy ISUP-GG. Diagnosis of ISUP GG≥2 or high-volume ISUP GG1 disease (defined as increase ≥33% volume disease from baseline biopsy) on confirmatory biopsy were considered as progression to csPCa. The predictive performance of the significant radiomic features was assessed both individually and in combination with a clinical predictive factor consisting of baseline clinicopathological parameters, with the aim of identifying a combined model with the highest overall performance.
MRI acquisition parameters
MRI examinations were performed at Fondazione Policlinico Universitario Agostino Gemelli IRCCS in Rome using a 1.5 T MRI scanner (GE Signa Exite, Little Chalfont, UK). All patients received intramuscular injection of anti- peristaltic agent unless contraindicated (20 mg hyoscine butylbromide, Buscopan®). Detailed MRI imaging protocol is reported in Table 1.
Administration of the contrast medium was not performed in 7/55 patients, so data from DCE-MRI were not considered for quantitative analysis because of the limited size of the sample and to give more homogeneity to the results.
Index lesion segmentation
Consensus of two radiologists with 11 and 4 years’ experience in genitourinary radiology reviewed the images and identified for each MRI examination one index lesion, defined as the lesion with the highest probability of malignance based on PIRADS v2.1.
Images were than compared to biopsy reports to check the concordance between imaging and pathological findings. In case of multiple lesions at MRI with the same Gleason Score at pathology, the largest one in the images was selected for the analysis. Thereafter, the index lesion (Gross Tumor Volume, GTV) was manually carefully contoured separately by two radiologists on the axial T2-WI HR, on the axial DWI images with a b-value of 1400 s/mm2 and on the relative ADC maps (Figure 2), by using radiotherapy Treatment Planning System (TPS) (Eclipse, Varian Medical Systems, Palo Alto, CA, USA).
Spatial planar resolution was 0.46 × 0.46 mm2 for T2-WI and 1.04 × 1.04 mm2 for DWI and ADC map. Afterward, for subsequent analysis the two readers re-segmented in consensus the images of the entire patient sample.
Table 1: MRI protocol adopted in the study.
Pulse Sequence |
Plane |
Repetition/ Echo Time (ms) |
Slice Thickness (mm) |
FOV (mm) |
Flip angle |
Duration |
Acquisition Matrix |
Sense Factor |
T2 TSE |
Axial |
4100/100 |
4 |
380 × 340 |
90° |
1’30’’ |
336 × 327 |
2 |
T2 TSE (HR) |
Sagittal |
2650/90 |
3.5 |
200 × 200 |
90° |
1’40’’ |
256 × 195 |
2 |
T2 TSE (HR) |
Coronal |
2500/100 |
3.5 |
160 × 160 |
90° |
3’25’’ |
288 × 228 |
2 |
T2 TSE (HR) |
Axial |
3730/90 |
3.5 |
400 × 490 |
90° |
4’10’’ |
288 × 240 |
2 |
DWI |
Axial |
4000/90 |
3.5 |
400 × 490 |
90° |
7’47’’ |
120 × 120 |
2 |
T1 DISCO Dynamic phase |
Axial |
2-Apr |
3.5 |
200 × 220 |
10° |
3’17’’ |
256 × 256 |
\ |
SSFSE |
Axial |
5000/80 |
3 |
400 × 350 |
90° |
51’ |
272 × 241 |
1.2 |
DWI= Diffusion Weighted Imaging; HR= High Resolution; SSFPE= Single Shot Fast Spin Echo; TSE= Turbo Spin Echo. |
Radiomic analysis
The DICOM files containing MR images and corresponding contours were exported from Eclipse and imported into a dedicated R software (Moddicom) designed to perform radiomic analysis [17,18]. Once imported, MR images were pre-processed by using a signal procedure normalization already reported in different experiences dealing to the MR images analysis [19].
Such approach consisted of normalising the signal intensity of the pixels within the GTV using as extremes the first and 99-th percentile of the GTV grey levels histogram and removing the pixel outside the levels. Radiomic analysis consisted in extracting 90 image features for each of three MR sequences delineated (T2-w, ADC and DWI). Radiomic features belonged to three families (statistical, textural and morphological) and were subjected to a standardisation initiative [20].
As regards the textural features, three grey level matrices were considered: run length (rlm), co-occurrence (cm) and
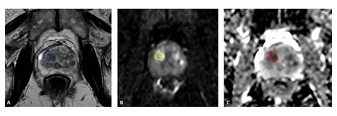
Figure 2: (A) Axial T2-weighted image shows a heterogeneous hypointense nodule with slightly blurred margins in the transitional zone of the right lobe of the prostate, at mid gland. The nodule appeared hyperintense on the Diffusion Weighted Images (b value 1500) (B) and hypointense on the ADC map images (C), and was as classified as PIRADS 3. The lesion was segmented separately by the two readers on the T2-weighted, DWI and ADC map images (respectively continuous blue line in A, yellow line in B and red line in C) in order to avoid registration errors as well as motion artifacts. size-zone (szm) matrices. The complete list of the radiomic features extracted is reported in the supplementary materials of similar experiences dealing with this topic and also in this manuscript [21]. A visual representation of the workflow used is reported in Figure 3.
Statistical analysis
The performance of the radiomic features in identifying patients who showed disease progression after at least two year from the diagnosis at the univariate analysis were evaluated by using the considering the Wilcoxon Mann Whitney (WMW) test or the t-test, depending on the normality of data distribution, which was previously assessed using the Shapiro-Wilk test [22].
The image features showing statistical significance at the univariate analysis were combined to all the other extracted radiomic features to create logistic regression models able to predict the disease progression.
Correlation among significant features was evaluated using the Pearson Correlation Coefficient (PCC), as reported in similar experiences [19].
Three predictive models were created:
- a pure radiomic model, combining the two most significant radiomic features an hybrid model, combining the most significant radiomic feature with the most significant clinical variable
- a 3 variables model combining the two previous models
To avoid overfitting, predictive models were created considering only two radiomic features and their predictive performance were evaluated considering the area (AUC) under the Receiver Operating Characteristic (ROC) curve and calculating sensitivity, specificity at the best threshold value, which was identified calculating the Youden Index (J) [22,23].

Figure 3: Visual representation of the workflow adopted for extraction of radiomic features. ACD= Apparent Diffusion Coefficient; DWI= Diffusion Weighted Images; GLCM= Gray-level Cooccurence Matrix; RLM= Run Length Matrix; ROC= Receiver Operating Characteristic; SZM= Size Zone Matrix; T2W= T2- Weighted.
The 95% confidence intervals (95%CI) for the ROC curves were calculated using the bootstrap method with 2000 iterations and the statistical significance of the difference in terms of AUC obtained among the three ROC curves was measured using the DeLong’s test. Not having at disposal an external cohort of validation, a three folds cross-validation was performed with 5 iterations to evaluate the robustness of the models proposed [24].
Results
The number of patients which did not respect the inclusion criteria were 19. This case-control study included 55 patients followed-up on the internal AS protocol from April 2015 to January 2020. Progression disease was observed in 22/55 cases, with an event rate of 40%.
The mean age of the study population was 69.1 ± 6.9 years old. All patients were followed-up for almost one year, and mean time-to-progression was 14.91 ± 6.2 months. Table2 presents the inter-group comparison of baseline clinic- pathological predictors of disease progression. Age was the only parameter discriminating between progressors and non- progressors, with a p value = 0.03. Clinical characteristics of the patients considered in the study were is reported in Table 2.
Radiomics analysis
A total of 270 radiomic features were extracted for each patient, considering 90 for each MRI sequence analysed (T2-w, DWI, ADC). At the univariate analysis, 10 radiomic features showed statistical significance (p<0.05 WMW analysis): five were extracted from DWI-MR images, five from T2w-MR images: the detailed results for the significant features are reported in Table 3.
No features extracted from the analysis of ADC-MR images showed significance in identifying responders at the univariate analysis. The only clinical feature showing statistical significance at the univariate analysis was the age, with a p-value of 0.021. The pure radiomic model consisted in the combination of two features extracted from the analysis of the DWI images: the correlation information 1 and the entropy. The radiomic model showed an AUC of 0.76 (95% CI of 0.62-0.89) in predicting progression to csPCa, with a sensitivity of 76.2% and a specificity of 66.7% at the best discriminative threshold (0.37).
The hybrid model consisted in the combination of the correlation information 1 and the age, with an AUC of 0.73 (95% CI of 0.58-0.87), a sensitivity of 68.2% and a specificity of 74.2% at the best threshold (0.44). The three variables model, obtained combining the two previous models, reported an AUC of 0.79 (95% CI of 0.66-0.92), a sensitivity of 62.0% and a specificity of 90.0% at the best threshold (0.56). The three ROC curves obtained with the corresponding IC levels are reported in Figure 4.
At the cross-validation, hybrid model showed an AUC of 0.69, pure radiomic model an AUC of 0.72 and three variables model an AUC of 0.76. No statistical significance was observed between the three models at the DeLong’s test (lowest p-value was 0.30 observed between hybrid and three variables model).
Discussion
This proof-of-concept study evaluated the role of radiomic analysis in identifying the baseline disease progression in patients with low risk PCa, who may be eligible for AS. In other words, we investigated the added value of radiomic features extracted from MRI target lesions. The purpose of radiomics in the context of PCa is to identify and propose new image-based biomarker in order to improve the risk- stratification, avoid unnecessary biopsies and reduce the risk of overtreatment. The integration of radiomics, imaging and clinicopathological data, could improve the prediction of tumor aggressiveness as well as guide clinical decisions and patient management [25,26].
Table 2: Clinical and histopathological characteristics of the patients included in the study. (mean ± standard deviation), divided in “progressors” (n= 22) and “non-progressors” (n=33). PIRADS v2 scores are evaluated in mpMRI performed at 9 months. ISUP= International Society of Urological Pathology; PIRADS= Prostate Imaging Reported And Data System; PSA= Prostate Specific Antigen.
Baseline predictor |
Progressors (n=22) |
Non-progressors (n=33) |
p value |
Age (years) |
71,55 ± 5.72 |
67,39 ± 7.21 |
0.03 |
PSA (ng/mL) |
6,27 ± 2.78 |
5,87 ± 2.43 |
0.58 |
Prostate volume (mL) |
62,50 ± 42.42 |
61,00 ± 44.68 |
0.9 |
PSA density |
0,13 ± 0.08 |
0,12 ± 0.07 |
0.63 |
PIRADS v2 score |
3,95 ± 0.49 |
3,61 ± 0.79 |
0.09 |
Biopsy ISUP GG 1 |
11 |
33 |
n.a. |
Biopsy ISUP GG ³ 2 |
11 |
0 |
n.a. |
Target lesion size (cm3) |
0,42 ± 0.31 |
0,43 ± 0.48 |
0.96 |
Time to progression (months) |
14,91 ± 6.23 |
n/a |
Table 3: Significant radiomic features at the univariate analysis. The textural features were calculated on the following grey-levels matrix:
Co-Occurence Matrix (CM), Run-Length Matrix (RLM), Size Zone (SZM).
MR sequence |
Feature Family |
Extended feature name |
Acronym |
p-value |
DWI |
Textural - CM |
Correlation information 1 |
cm.info.corr.1 |
0,021 |
DWI |
Statistical |
Entropy |
Entropy |
0,027 |
DWI |
Textural - RLM |
Grey-levels non uniformity |
rlm.glnu |
0,030 |
DWI |
Textural - CM |
Contrast |
cm.contrast |
0,038 |
DWI |
Textural- SZM |
Grey-levels non uniformity |
szm.glnu |
0,038 |
T2 |
Textural - CM |
Correlation information 2 |
cm.info.corr.2 |
0,027 |
T2 |
Textural - CM |
Sum variance |
cm.sum.var |
0,032 |
T2 |
Textural - CM |
Cluster tendency |
cm.clust.tend |
0,032 |
T2 |
Textural - CM |
Joint variance |
cm.joint.var |
0,038 |
T2 |
Textural - CM |
Cluster prominence |
clust.prom |
0,039 |
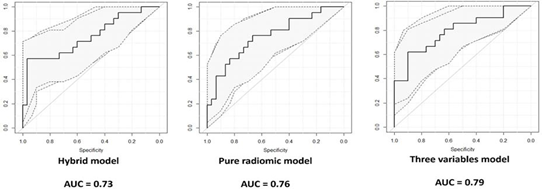
Figure 4 : ROC curve of the three predictive models with corresponding 95% confidence interval.
In our study, the radiomic analysis identified ten features that showed a statistically significant differential expression between patients who have progressed to clinically significant disease and not. Therefore, we created three predictive radiomic models of progression-risk and investigated the add value of radiomic analysis in patients suitable for AS. The best predictive model was an hybrid model combining two radiomic features extracted from DWI images and the age, reaching an AUC of 0.79 on the training set and an AUC of 0.76 at the cross-validation.
Several studies have already reported the potential role of radiomic analysis in PCa detection [10,27], differentiation of small neoplastic lesions from benign ones [28], assessment of lesion aggressiveness [29], local staging and pre-treatment assessment [30], detection of recurrence or prediction of treatment outcome [31].
The results of this study, demonstrated that radiomic analysis may allow evaluating the baseline progression risk of the target lesion, suggesting a possible role for MRI- derived radiomics as an additional risk-stratification tool to triage patients suitable for AS. Radiomic analysis may help to overcome the limits of MRI and clinicopathological parameters in identifying patients with low risk progression, who can be candidates to AS. This is an important added value considering the increasing use of AS. A recent analysis utilizing the CAPSURE database, highlighted that the option of AS ranged from 6.7% to 14.3% since 1990 through 2009, but increased sharply between 2010 to 2013 when 40.4% of low risk PCa patients had opted for this approach [32]. However, it is expected that up to 30-50% of patients with low-risk disease would be upgraded to csPCa during the next 5 year or upstaged at the time of radical prostatectomy (RP) [33,34]. Therefore, baseline risk stratification in patients labeled as “low risk” is of paramount importance. A progression predictive model has never entered routine clinical practice due to their low predictive accuracy and poor performance with external validation [15]. The role of mpMRI in identifying patients suitable for AS was expanded over the years and a lot of studies have confirmed the ability of mpMRI to better identify men at risk of immediate re- staging after initial diagnosis [35]. Despite the introduction of the Prostate Imaging-Reporting and Data System (PI-RADS) v2.1 to simplify terminology and mpMRI report content, subjectivity and interobserver variability still exist [36]. A multicenter study conducted on interobserver reproducibility of the PI-RADS v2 lexicon conducted by Rosenkrantz et al. [37] found that only experienced radiologists achieved moderate reproducibility for PI-RADS v2.1 and the agreement was better in PZ than in TZ [37]. As reported by Greer et al. [38], MRI-derived radiomic features may improve the inter- reader agreement by creation of machine learning model [38]. To the best of our knowledge, only few studies investigated the role of radiomics on prostate MRI in patients eligible for AS. The study of Algohary et al. [14] evaluated if radiomics features on bi-parametric MRI could identify csPCa in highly challenging cases where there was a discordance between PI- RADS and histopathologic findings for PCa patients on AS. They found 7 T2w-MRIs and 3 ADC-MRIs features which showed significant differences between benign and malignant lesions and concluded that radiomic analysis could help in identifying the presence and absence of clinically significant disease in AS patients. However, this study excluded PI- RADS 3 lesions, which which are indeterminate and pose a major clinical management challenge. [39,40]. Unlike Algohary’s work, we included PI-RADS 3 lesions believing that radiomic derived features might have an added value to convential prostate MRI improving the interpretation of these indeterminate lesions, in order to reduce inter-reader variability. Sushentsev et al. [15] recently evaluated the added value of MRI-derived radiomic features to standard of care clinical parameters for improving baseline prediction of PCa progression in AS patients. They found a similarly moderate predictive performance by T2W-MRIs and ADC- MRIs derived radiomic model alone, as well as for their combination. Moreover, the best predictive performance was achieved combining T2w-derived radiomic features with PSA and PSAD (AUC=0.75; 95% CI, 0.636-0.862).
Similarly, we created multiple predictive models including the best radiomic features and clinicopathological predictors. We found the best performance model combining the two best DWI-derived radiomics. This performance slightly increased by combining these DWI-derived radiomics with the age, as showed in Figure 2. In our study we included T2w, DWI and ADC MR sequences, while the other studies analyzed only T2w and ADC sequences. We believe that the information’s provided by DWI and ADC are quite different and needed to be both included in our analysis. DWI images quantify the diffusion of water molecules in the tissue, while ADC shows the rate of variation of the DWI signal intensity with respect to a change in b-values. However, in our study no features extracted from the analysis of ADC showed the ability of predicting the progression in AS patient.
This study has several limitations. This is a retrospective study and data were collected from a single center. Moreover, we didn’t consider DCE sequences, because the administration of the contrast medium was not performed in 7/55 patients.
For this reason that data from DCE-MRI were not considered for quantitative analysis in order to give more homogeneity to the results. In the future, a multicenter prospective analysis should be carried out to validate these results.
Conclusion
The case series and systemic review of the literature provide a few learning points.
−Not every infected pneumatocele or lung cyst requires
surgical intervention
−Persistent air-leak, associated pneumothorax and
haemothorax suggest more urgent surgical intervention
−Secondary infection of the pneumatocele not-responding to extended antibiotic therapy is a potential life threatening condition and should be considered for surgical resection. Prompt surgical approach may be lifesaving in patients in the critical clinical stage
−In patients with newly discovered pneumatocele after recovering from COVID-19, repeated imaging in 4-6 weeks may prevent further complications
−Second imaging in a prone position could help to
distinguish a pneumothorax from a pneumatocele
References
- Sung H, Ferlay J, Siegel RL. Global cancer statistics 2020: GLOBOCAN estimates of incidence and mortality worldwide for 36 cancers in 185 countries. CA Cancer J Clin 71(3) (2021): 209-249.
- Neal DE, et al. Ten-year mortality, disease progression, and treatment-related side effects in men with localised prostate cancer from the ProtecT randomised controlled trial according to treatment Eur. Urol. 77 (2020): 320-330.
- Wilt TJ, et Follow-up of prostatectomy versus observation for early prostate cancer. N. Engl. J. Med 377(2) (2017): 132-142.
- Carlsson S, Benfante N, Alvim R et Long-term outcomes of active surveillance for prostate cancer the Memorial Sloan Kettering Cancer Center experience. J Urol 203 (2020): 1122-1127.
- Van Hemelrijck Reasons for discontinuing active surveillance: Assessment of 21 centres in 12 countries in the movember GAP3 consortium. Eur. Urol 75 (2019): 523-531.
- Sierra PS, Damodaran S, Jarrard Clinical and pathologic factors predicting reclassification in active surveillance cohorts. Int. Braz J Urol 44 (2018): 440-451.
- Turkbey B, Rosenkrantz AB, Haider Prostate Imaging Reporting and Data System Version 2.1: 2019 Update of Prostate Imaging Reporting and Data System Version 2. Eur Urol 76 (2019): 340-351.
- Min X, Li M, Dong D, et Radiology.115 (2019): 16-21.
- Parmar C, Grossmann P, Bussink J. Machine learning methods for quantitative radiomic biomarker. Sci Rep 5(2015): 13087.
- Wang J, Wu CJ, Bao ML et al. Machine learning-based analysis of MR radiomics can help to improve the diagnostic performance of PI-RADS v2 in clinically relevant prostate cancer. Eur Radiology 27 (2017): 4082-
- Zhang GM, Han YQ, Wei JW, et Radiomics Based on MRI as a Biomarker to Guide Therapy by Predicting Upgrading of Prostate Cancer From Biopsy to Radical Prostatectomy. Imaging 52 (2020): 1239-1248.
- Losnegård A, Reisæter LAR, Halvorsen OJ, et Magnetic resonance radiomics for prediction of extraprostatic extension in non-favorable intermediate- and high-risk prostate cancer patients. Radiology 61 (2020): 1570-1579.
- Litjens GJS, Elliott R, Shih Computer-extracted features can distinguish noncancerous confounding disease from prostatic adenocarcinoma at multiparametric MR imaging. Radiology 278 (2016): 135-145.
- Algohary A, Viswanath S, Shiradkar Radiomic features on MRI enable risk categorization of prostate cancer patients on active surveillance: preliminary findings. J Magn Reson Imaging 10(2018): 1002.
- Nikita Sushentsev, Leonardo Rundo, Oleg Blyuss, et al. MRI-derived radiomics model for baseline.11(1)(2021):
- Epstein JL, Walsh PC, Carmichael M, et al. Pathological and clinical findings to predict tumour extent of non- palpable (stage T1c) prostate cancer. JAMA 271 (1994): 368-374.
- Gatta R, Vallati M, Dinapoli N, et al. Towards a modular decision support system for radiomics: A case study on rectal Artif Intell Med 96(2018): 145-153.
- Dinapoli N, Alitto AR, Vallati M, et al. Moddicom: a complete and easily accessible library for prognostic evaluations relying on image features. Conf Proc IEEE Eng Med Biol Soc 10 (2015): 771-774.
- Cusumano D, Meijer G, Lenkowicz J, et A field strength independent MR radiomics model to predict pathological complete response in locally advanced rectal cancer. Radiol Med 126(3)(2020): 421-429.
- Zwanenburg A, Vallières M, Abdalah MA, et al. The Image Biomarker Standardization Initiative: Standardized Quantitative Radiomics for High-Throughput Image- based Radiology 295 (2020):328-338.
- Cusumano D, Boldrini L, Yadav P, et External Validation of Early Regression Index (ERITCP) as Predictor of Pathologic Complete Response in Rectal Cancer Using Magnetic Resonance-Guided Radiation Therapy. Int J Radiat Oncol Biol Phys 108(5)(2020): 1347-1356.
- Taylor J. Introduction to Error Analysis, the Study of Uncertainties in Physical Measurements, 2nd Published by University Science Books, 648 Broadway, Suite 902, New York, 5(1997).
- Cusumano D, Catucci F, Romano A, et Evaluation of an Early Regression Index (ERITCP) as Predictor of Pathological Complete Response in Cervical Cancer: A Pilot-Study. Applied Sciences 10(22)(2020): 8001.
- Cusumano D, Boldrini L, Yadav P, et Delta Radiomics Analysis for Local Control Prediction in Pancreatic Cancer Patients Treated Using Magnetic Resonance Guided Radiotherapy. Diagnostics 11(2021):72.
- Kumar V, Gu Y, Basu S, et Radiomics: the process and the challenges. Magn Reson Imaging 30 (2012):1234- 1248.
- Lambin P, Leijenaar RTH, Deist TM, et al. Radiomics: the bridge between medical imaging and personalized Nature Reviews Clinical Oncology 14 (2017): 749-762.
- Ginsburg SB, Algohary A, Pahwa S, et al. Radiomic features for prostate cancer detection on MRI differ between the transition and peripheral zones: preliminary findings from a multi-institutional study. J Magn Reson Imaging 46(1)(2017): 184-193.
- Wibmer A, Hricak H, Gondo T. Haralick texture analysis of prostate MRI: utility for differentiating non-cancerous prostate from prostate cancer and differentiating prostate cancers with different Gleason scores. Eur Radiology 25 (2019):2840 -2850.
- Varghese B, Chen F, Hwang D, et al. Objective risk stratification of prostate cancer using machine learning and radiomics applied to multiparametric magnetic resonance images 9(1)(2019): 1570.
- Stanzione A, Cuocolo R, Cocozza S, et Detection of extraprostatic extension of cancer on biparametric MRI combining texture analysis and machine learning: preliminary results. Acad Radiology 26(10) (2019):1 -7.
- Gu Y, Lin X, Kapoor A, et al. Attempt to predict early recurrence of prostate cancer following prostatectomy through machine learning. AME Med J 3(2018): 96.
- Cooperberg MR, Carroll PR. Trends in Management for Patients With Localized Prostate Cancer, 1990-2013. JAMA 314 (2015): 80-82.
- Pinthus JH, Witkos M, Fleshner NE, et Prostate cancers scored as Gleason 6 on prostate biopsy are frequently Gleason 7 tumors at radical prostatectomy: implication on outcome. J Urol 176 (2006): 979-984.
- Epstein JI, Feng Z, Trock BJ, et Upgrading and downgrading of prostate cancer from biopsy to radical prostatectomy: incidence and predictive factors using the modified Gleason grading system and factoring in tertiary grades. Eur Urol 61 (2012):1019-1024.
- Turtle D, et al. Progression and treatment rates using an active surveillance protocol incorporating image-guided baseline biopsies and multiparametric magnetic resonance imaging monitoring for men with favourable-risk prostate 122(1)(2018): 59-65.
- Turkbey B, Rosenkrantz AB, Haider MA, et al. Prostate Imaging Reporting and Data System 42(1) (2017): 278-289.
- Update of Prostate Imaging Reporting and Data System Version Eur Urol 76 (2019): 340.
- Rosenkrantz AB, Ginocchio LA, Cornfeld D, et Interobserver Reproducibility of the PI-RADS Version 2 Lexicon: A Multicenter Study of Six Experienced Prostate Radiologists. Radiology 280 (2016): 793-804.
- Greer MD, Lay N, Shih JH, et Computer-aided diagnosis prior to conventional interpretation of prostate mpMRI: an international multi-reader study. Eur Radiol 28 (2018):4407-4417.
- Cornud Bi-parametric prostate MRI before biopsy: Yes, but only if you deserve it. Diagn Interv Imaging 01 (2020): 191-192.
- Brancato V, Aiello M, Basso L, et Evaluation of a multiparametric MRI radiomic-based approach for stratification of equivocal PI-RADS 3 and upgraded PI-RADS 4 prostatic lesions. Sci Rep 11 (2021): 643.