Identification of Promising Antagonists of the Tumor Microenvironment Immunosuppressive Adenosine 2A Receptor through a Pharmacoinformatics- Based Approach
Article Information
Soumaya Rafii1, Yassine El Ghallab2, Amina Ghouzlani1, Sarah Kandoussi1, Abdallah Badou1*
1Cellular and Molecular Pathology Laboratory, Faculty of Medicine and Pharmacy of Casablanca, Hassan II University, Casablanca, Morocco
2Laboratory of Drugs Sciences, Biomedical Research and Biotechnology, Faculty of Medicine and Pharmacy, Hassan II University, Casablanca, Morocco
*Corresponding author:Abdallah Badou, Cellular and Molecular Pathology Laboratory, Faculty of Medicine and Pharmacy of Casablanca, Hassan II University, Casablanca, Morocco.
Received: 19 September 2021; Accepted: 06 October 2021; Published: 25 October 2021
Citation: Soumaya Rafii, Yassine El Ghallab, Amina Ghouzlani, Sarah Kandoussi, Abdallah Badou. Identification of Promising Antagonists of the Tumor Microenvironment Immunosuppressive Adenosine 2A Receptor through a Pharmacoinformatics-based approach. Journal of Bioinformatics and Systems Biology 4 (2021): 122-139.
Share at FacebookAbstract
Tumor cells can reverse the immune system's control through secretion or surface expression of several molecules such as immune checkpoint inhibitors. Blocking these immune checkpoint molecules could reactivate the immune system against cancer cells. In the present study, we focused on the use of natural substance-derived molecules and their ability to inhibit the adenosine 2A receptor (A2AR). Eightyeight molecules were selected using the nutrigenomeDB and fingerprint method, then evaluated according to their absorption, distribution, metabolism, excretion, and toxicity (ADMET) properties using admetSAR tools. Furthermore, structural study of “molecule-A2AR” binding sites affinity and druggability were also assessed using autodock vina, discovery studio visualizer, and Cavityplus. Our results identified two molecules, suregadolideA and suremulolA, which exhibited suitable ADMET properties. These molecules bound to A2AR with high affinity and showed an important drug score. These molecules will now be tested experimentally on cells to check whether they could be used as potential A2AR antagonists, able to overcome the immune system inhibition, leading to the control of tumor progression.
Keywords
Immune checkpoint inhibitors; Natural molecules; A2AR expression; A2AR antagonists; ADMET; Docking; Druggability
Immune checkpoint inhibitors articles; Natural molecules articles; A2AR expression articles; A2AR antagonists articles; ADMET articles; Docking articles; Druggability articles.
Immune checkpoint inhibitors articles Immune checkpoint inhibitors Research articles Immune checkpoint inhibitors review articles Immune checkpoint inhibitors PubMed articles Immune checkpoint inhibitors PubMed Central articles Immune checkpoint inhibitors 2023 articles Immune checkpoint inhibitors 2024 articles Immune checkpoint inhibitors Scopus articles Immune checkpoint inhibitors impact factor journals Immune checkpoint inhibitors Scopus journals Immune checkpoint inhibitors PubMed journals Immune checkpoint inhibitors medical journals Immune checkpoint inhibitors free journals Immune checkpoint inhibitors best journals Immune checkpoint inhibitors top journals Immune checkpoint inhibitors free medical journals Immune checkpoint inhibitors famous journals Immune checkpoint inhibitors Google Scholar indexed journals Natural molecules articles Natural molecules Research articles Natural molecules review articles Natural molecules PubMed articles Natural molecules PubMed Central articles Natural molecules 2023 articles Natural molecules 2024 articles Natural molecules Scopus articles Natural molecules impact factor journals Natural molecules Scopus journals Natural molecules PubMed journals Natural molecules medical journals Natural molecules free journals Natural molecules best journals Natural molecules top journals Natural molecules free medical journals Natural molecules famous journals Natural molecules Google Scholar indexed journals A2AR expression articles A2AR expression Research articles A2AR expression review articles A2AR expression PubMed articles A2AR expression PubMed Central articles A2AR expression 2023 articles A2AR expression 2024 articles A2AR expression Scopus articles A2AR expression impact factor journals A2AR expression Scopus journals A2AR expression PubMed journals A2AR expression medical journals A2AR expression free journals A2AR expression best journals A2AR expression top journals A2AR expression free medical journals A2AR expression famous journals A2AR expression Google Scholar indexed journals A2AR antagonists articles A2AR antagonists Research articles A2AR antagonists review articles A2AR antagonists PubMed articles A2AR antagonists PubMed Central articles A2AR antagonists 2023 articles A2AR antagonists 2024 articles A2AR antagonists Scopus articles A2AR antagonists impact factor journals A2AR antagonists Scopus journals A2AR antagonists PubMed journals A2AR antagonists medical journals A2AR antagonists free journals A2AR antagonists best journals A2AR antagonists top journals A2AR antagonists free medical journals A2AR antagonists famous journals A2AR antagonists Google Scholar indexed journals Docking articles Docking Research articles Docking review articles Docking PubMed articles Docking PubMed Central articles Docking 2023 articles Docking 2024 articles Docking Scopus articles Docking impact factor journals Docking Scopus journals Docking PubMed journals Docking medical journals Docking free journals Docking best journals Docking top journals Docking free medical journals Docking famous journals Docking Google Scholar indexed journals Druggability articles Druggability Research articles Druggability review articles Druggability PubMed articles Druggability PubMed Central articles Druggability 2023 articles Druggability 2024 articles Druggability Scopus articles Druggability impact factor journals Druggability Scopus journals Druggability PubMed journals Druggability medical journals Druggability free journals Druggability best journals Druggability top journals Druggability free medical journals Druggability famous journals Druggability Google Scholar indexed journals Tumor Microenvironment articles Tumor Microenvironment Research articles Tumor Microenvironment review articles Tumor Microenvironment PubMed articles Tumor Microenvironment PubMed Central articles Tumor Microenvironment 2023 articles Tumor Microenvironment 2024 articles Tumor Microenvironment Scopus articles Tumor Microenvironment impact factor journals Tumor Microenvironment Scopus journals Tumor Microenvironment PubMed journals Tumor Microenvironment medical journals Tumor Microenvironment free journals Tumor Microenvironment best journals Tumor Microenvironment top journals Tumor Microenvironment free medical journals Tumor Microenvironment famous journals Tumor Microenvironment Google Scholar indexed journals Pharmacoinformatics articles Pharmacoinformatics Research articles Pharmacoinformatics review articles Pharmacoinformatics PubMed articles Pharmacoinformatics PubMed Central articles Pharmacoinformatics 2023 articles Pharmacoinformatics 2024 articles Pharmacoinformatics Scopus articles Pharmacoinformatics impact factor journals Pharmacoinformatics Scopus journals Pharmacoinformatics PubMed journals Pharmacoinformatics medical journals Pharmacoinformatics free journals Pharmacoinformatics best journals Pharmacoinformatics top journals Pharmacoinformatics free medical journals Pharmacoinformatics famous journals Pharmacoinformatics Google Scholar indexed journals elimination and toxicity articles elimination and toxicity Research articles elimination and toxicity review articles elimination and toxicity PubMed articles elimination and toxicity PubMed Central articles elimination and toxicity 2023 articles elimination and toxicity 2024 articles elimination and toxicity Scopus articles elimination and toxicity impact factor journals elimination and toxicity Scopus journals elimination and toxicity PubMed journals elimination and toxicity medical journals elimination and toxicity free journals elimination and toxicity best journals elimination and toxicity top journals elimination and toxicity free medical journals elimination and toxicity famous journals elimination and toxicity Google Scholar indexed journals
Article Details
1. Introduction
To protect the human body against various dangers, the immune system plays a crucial role in maintaining intrinsic homeostasis through various molecules such us immune checkpoint inibitors [1]. However, these can be cooped by the tumor to escape the immune system's surveillance. Therefore, the immune cells, which are ready to act on cancer cells, find themselves controlled by different molecular pathways, inhibiting their activation and their effector functions [1]. Among several checkpoint molecules, we consider that adenosine 2A receptor (A2AR) is known to be involved in tumor progression [2,3]. It could protect cancerous tissues from immune clearance via inhibiting T cell response in head and neck squamous cell carcinoma and melanoma [2,3]. Activation of A2AR on T cells prevented TCR-mediated transcription factor AP-1 signaling and promoted T cell tolerance [4]. In patients with glioma, the A2AR/CD73/CD39 pathway was the most frequently expressed, followed by the PD-1 pathway [5]. In sarcoma, the blockade of the A2AR on adoptively transferred T cells by synthetic A2AR antagonist led to higher levels of IFN-γ secretion by tumor-infiltrating CD8+ T cells [6]. In order to target immune checkpoint molecules, several studies assessed the ability of therapeutic natural molecules. One objective is to avoid adverse side effects that can occur with antibody-based immunotherapy and, another major objective is to make this type of therapy more accessible to most patients [7,8]. Drug discovery takes place over a long time, taking around 20 years. Even with the increase in their expenses in the last few years, the number of new drugs introduced into the market has been steadily decreasing. It is a consequence of preclinical and clinical safety issues [9]. In silico studies play an essential role in solving this problem. They are being used for an early-stage prediction of potential safety issues, increasing the drug discovery success rate, and reducing costs linked with developing a new drug [9]. In silico studies are primarily concerned with the properties of absorption, distribution, metabolism, elimination and toxicity (ADMET) of the drug in the body in which the drug will be administered. These characteristics define the stages in the fate of a drug in the body [10]. Also, molecular docking is an important tool in assessing a molecule's affinity with a receptor and determining the function of their interaction via binding sites [11]. In this study, we attempted to identify potential molecules capable of blocking the A2AR. To do this, we started with a screening of 88 molecules according to their ADMET characteristics. Subsequently, we assessed the affinity to the A2AR and evaluated the function and druggability upon binding site occupancy. Our results revealed two A2AR-targeting molecules with interesting features, suregadolideA and suremulolA. These two molecules showed no cellular toxicity and exhibited appropriate ADME properties. Furthermore, these two molecules bound to A2AR with high affinity and showed an important drug score.
2. Methodology
2.1 Searching for natural molecules, which could bind to and inhibit A2AR
NutriGenomeDB exploratory and analytical platform was used for the choice of natural molecules that could bind to and inhibit A2AR [12]. This platform defines specific genes by differential expression analysis of nutrigenomic experiments, performed on human cells available in the Gene Expression Omnibus (GEO). Each experiment is defined by a set of genes, representing approximately 10% of differentially expressed genes, sorted by differential expression levels. The modulation of expression levels of the A2AR gene by natural molecules were obtained in tabular form, showing ten independent experiments. The significance is evaluated according to adjusted.p-value(<0.05). The platform is available on http://nutrigenomedb.org/search. Analogs of natural molecules were collected from articles and using natural product activity and species source database (NPASS) [13]. This search for similar structures was performed based on fingerprint methods (threshold≥0.70). Graphics were generated by GraphPad prism6.
2.2 ADMET prediction of natural molecules
The absorption, distribution, metabolism, and toxicity of natural molecules determine their pharmacokinetic profile. In this study, the prediction of ADMET characteristics was performed using the admetSAR prediction tool, based on SMILES structures of the molecules of interest [14]. Graphics were generated by GraphPad prism6.
2.3Ligands and receptor preparation
ChemOffice Professional version 18.0.0.231(http://www.cambridgesoft.com/) was used to generate a better ligand conformation for docking in Protein Data Bank(PDB) file format, the drawed3D geometry structures of natural molecules and were optimized to minimum energy (Allinger 1977). All rotatable bonds present in the ligands were treated as non-rotatable. The Gasteiger charges were added to the ligand atoms before docking (Gasteiger and Marsili 1980). The crystal structure of A2AR (PDB ID: 6gdg) was retrieved from RCSB Protein Data Bank (https://www.rcsb.org/). All water molecules and heteroatoms were removed from the crystal structures, and polar hydrogen atoms were added using Discovery Studio Visualizer v19.1.0.18287 (https://www.3ds.com/) Grid box preparation and docking. Docking experiments were conducted with selected natural molecules using A2AR as specific target. Grid box parameters were set by using the graphic user interface AutoDock Tools (ADT), version 1.5.6, of Molecular Graphics Laboratory(MGL) software packages of the Scripps Research Institute (Michel et al. 2011). The center grid box was set at x = 78.122, y = 89.064, and z = 85.083 points. The number of points in x, y, and z dimensions was adjusted to 84, 120, and 76. The spacing value between grid points was 1Å. The molecular docking program AutoDockVina (version 1.1.2) (Trott and Olson 2010) was used to perform the docking experiment. The Lamarkian Genetic Algorithm was used to explore the best conformation space for the ligand with a population size of 150 individuals during the docking process. The maximum number of generations was set at 27000, and the evaluation one was set at 2500000. Other parameters were fixed as default.
2.4 Visualisation of binding sites
Using the Discovery Studio Visualization software, we started by visualizing the results using the receptor and ligands in PDB.qt format. Then we evaluated the interactions between the A2AR and each ligand to calculate the length of the bonds. Finally, we labelled the interacting residues in order to determine the amino acid region on which the ligand would bind.
2.5 Study of druggability of natural molecules-A2AR binding sites
Identification of possible binding sites in receptor structure enables us to analyze and classify natural molecules through their druggability. Cavityplus is a better tool for precise and robust protein cavity detection and functional analyses [15]. A2AR are uploaded in the PDB format and analyzed by CAVITY with default parameters to detect potential ligand-binding sites. The CAVITY module was performed with default parameters and ‘no Ligand’ mode.
3. Results and Discussion
3.1 EnglerinA negatively modulates the expression of the A2AR gene
To explore the best natural substances able to downregulate the expression of A2AR, we used NutriGenomeDB platform, the first nutrigenomics data exploration platform that studies the impact of nutrients and bioactive food molecules on gene expression. The present study showed only the experiments where the A2AR gene appears in the gene subset defined by the analyzed nutrigenomic experiments. Nutrigenomic gene sets were defined by the top 10% differentially expressed genes sorted by their differential expression level (figure1). The results showed that A2AR was in the top 10% genes only in ten platform experiments. In addition, englerinA was the only compound, which showed a statistically significant decrease on A2AR gene expression (log2 fc = -1; p-value = 0.001 and adjusted p-value = 0.042). The analysis was carried out on renal cell carcinoma (A-498) after 20 hours of treatment with EnglerinA. In the same sense, we observed a decreasing trend of A2AR expression in human myotubes, 24 hours after treatment with eicosapentaenoic acid (log2 fc = -0.3) and in prostate cancer cells 24 hours after treatment with 6-acetoxy-anopterine (6AA) (log2 fc=-0.3). Supplementary table 1 contains more details on each experiment.

Figure 1:EnglerinA negatively modulates the expression of the A2AR gene
3.2 EnglerinA toxicity prediction:
Many molecules have favorable properties but still, lead to failure when administered to a living organism due to theirtoxicities. Using admetSAR tools based on a SMILES structure, we were able to identify a prediction of the toxicity of englerinA (table 1). Results of toxicity prediction revealed that englerinA could induce Human either-a-go-go-related gene (hERG) inhibition.
![]() |
Table1: Toxicity profile of antagonists potential molecules.
3.3 Screening of analogs
The predicted toxic effect of englerinA on the heart prompted us to look for other similar ligands which exhibit less toxicity on the body. For this reason, we analyzed other studies on the NPASS platform by fingerprint method to find similar natural structures and analogs of englerinA. To widen the field of candidate molecules, we have also analyzed similar eicosapentanoic acid molecules and 6-acetoxy-anopterine, which also tended to decrease the A2AR gene (a total of 88 natural molecules was evaluated). Since absorption, distribution, metabolism, excretion, and toxicity (ADMET) characteristics are essential in developing and designing therapeutic substances, the violation of these characteristics causes several drugs failure and renders them ineffective despite their strong affinity to the target of interest. According to ADMET characteristics, we started the selection based on their toxicity profile which includes genotoxicity, mutagenicity, hepatotoxicity, side effects on the heart, and carcinogenicity (table 1).
Natural molecules |
HIA |
Oral bioavailivility |
Caco2cells |
Beilschmiedic Acid L |
+ |
- |
- |
Beilschmiedic Acid K |
+ |
- |
- |
CHEMBL251681 |
+ |
- |
- |
Beilschmiedic Acid I |
+ |
- |
- |
Beilschmiedic Acid M |
+ |
- |
- |
Phyllaemblic Acid Methy Ester |
+ |
- |
- |
Longumoside B |
- |
- |
- |
Visartiside D |
- |
- |
- |
Eutigoside C |
+ |
- |
- |
Gleditschiaside A |
+ |
- |
- |
CHEMBL2436431 |
- |
- |
- |
Eicosapentaenoicacid |
+ |
+ |
- |
Arachidonicacid |
+ |
+ |
+ |
Doconexent |
+ |
+ |
- |
Octadeca-9,12,15-Trienoic Acid |
+ |
- |
+ |
(E)-Pentadec-10-En-6,8-Diynoic Acid |
+ |
- |
+ |
Dicranin |
+ |
+ |
- |
Linolenic Acid |
+ |
- |
+ |
adrenicacid |
+ |
- |
- |
Docosapentaenoic Acid |
+ |
- |
- |
Oleandrigenin |
+ |
+ |
- |
Coroglaucegenin |
+ |
+ |
- |
3-oxo-8beta,14beta-epoxy-ent-abieta-11,13(15)-dien-16,12-olide |
+ |
+ |
+ |
cardinolide |
+ |
+ |
+ |
stearidonicacid |
+ |
+ |
- |
suregadolide A |
+ |
+ |
+ |
suregadolide B |
+ |
+ |
+ |
suremulol A |
+ |
+ |
+ |
Albopilosin B |
+ |
- |
- |
Xindongnin B |
+ |
- |
- |
Gitoxigenin |
+ |
+ |
- |
Digoxigenin |
+ |
- |
- |
Acovenosigenin A |
+ |
- |
- |
Sarmentogenin |
+ |
+ |
- |
Digitoxigenin |
+ |
+ |
+ |
Deacetylvitexilactone |
+ |
- |
+ |
*HIA: Human intestinal absorption.
Table 2: Absorption prediction of selected natural molecules
Our results showed that among the 88 analyzed molecules, only 36 were not toxic. The 36 selected molecules were then evaluated according to their intestinal and caco2 cell absorption capacity and their oral bioavailability (table2). However, we were able to select only eight substances capable of being absorbed by the intestine and coco2 cells and were therefore orally bioavailable. The latest sort focuses on the capacity of distribution and metabolism of thesetop molecules. Distribution was assessed by the ability to cross the blood-brain barrier, and to eventually impact P-glycoprotein (PGP) and plasma protein binding (PPB) levels (table 3). Only substances with the capacity to cross the BBB and were neither substrates noinhibitors of PGP and having a PPB rate <0.9 were retained. Results showed that onlyarachidonic acid, cardenolide, suregadolideA, suregadolideB, and suremulolA, showed a good absorption rate. Arachidonic acid, cardenolide, suregadolideA, suregadolideB and suremulolA, exhibited high probabilities of intestinal absorption, anability to cross the BBB (greater than 90%), an absorption by caco-2 cells and a suitable oral bioavailability (greater than 51%) (figure2). In order to evaluate the metabolism of these five molecules, we analyzed two parameters: substrates and inhibitors of CYP enzymes (table3). Our results demonstrated that suremulolA is a substrate for two cytochromes (CYP) enzymes, CYP3A4 and CYP2C9. However, the other four substances are substrates for only one CYP enzyme. Otherwise, suremulolA does not induce CYP inhibition, unlike arachidonic acid and suregadolideB which induce CYP1A2 inhibition.
![]() |
* BBB: Blood- brain barrier; Pgp:p-glycoprotein; (s): Substrate; (I): Inhibitor; PPB: Plasma protein binding.
Table 3: Distribution and metabolism prediction of selected molecules
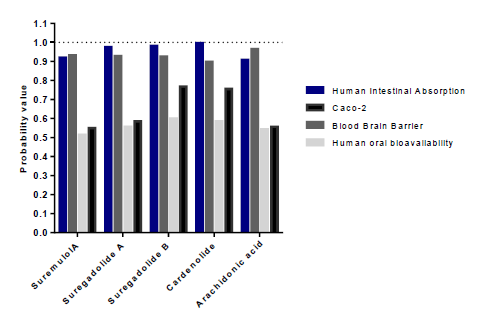
Figure 2:Probabilities of absorption and distribution of natural molecules.
3.4 Assessment of the affinity of the sorted molecules for A2AR
To assess the affinity of arachidonic acid, cardenolide, suregadolideA, suregadolideB and suremulolA for the A2AR. AutoDock Vina software has been used for this purpose. Affinity for the protein was estimated through vina score, which is also known as docking energies (Kcal/mol). Caffeine has been reported to show potent effects on the inhibition of A2AR (table 4), with an energy score ranging from -7.7 to -9.9 Kcal/mol. Furthermore, the classification of molecules with the highest energy score to the lowest was described, arachidonic acid with -7.7 Kcal / mol, cardenolide with -8.3Kcal / mol, suregadolideB with -9.2 Kcal / mol, suremulolA with - 9.8 Kcal / mol and suregaolideA with -9.9 Kcal / mol. These molecules showed an energy score inferior to that of the control, caffein (-6.7 Kcal / mol), indicating high affinity to A2AR.
Natural molecules |
Binding Energy (Kcal/mol) |
Caffein |
−6.7 |
Arachidonicacid |
−7.7 |
Cardenolide |
−8.3 |
Suregadolide B |
−9.2 |
Suremulol A |
−9.8 |
Suregadolide A |
−9.9 |
Table 4: Binding energy values of the selected natural molecules
Structural analysis of “molecules-A2AR” binding sites and druggability
Such as ADMET properties and affinity prediction of molecules, determination of binding sites is of particular interest in designing a therapeutic process. Indeed, this allows a prediction of potential druggability of distinct molecules. Therefore, using the discovery studio visualizer, each substance's binding sites on the A2AR were identified. Each binding site is made up of aminoacid residues (figure 3). Our results indicated that arachidonic acid (a),cardenolide (b), suremulolA (e) and suregadolideB (d) have one conventional hydrogen bond interaction with aminoacids. However, suregadolideA(c) has three conventional hydrogen bonds. As control, caffeine(f) presented 2 hydrogen bonds. Other important interactions such as hydrophobic interaction, Pi-alkyl, alkyl interactions were observed for all molecules.
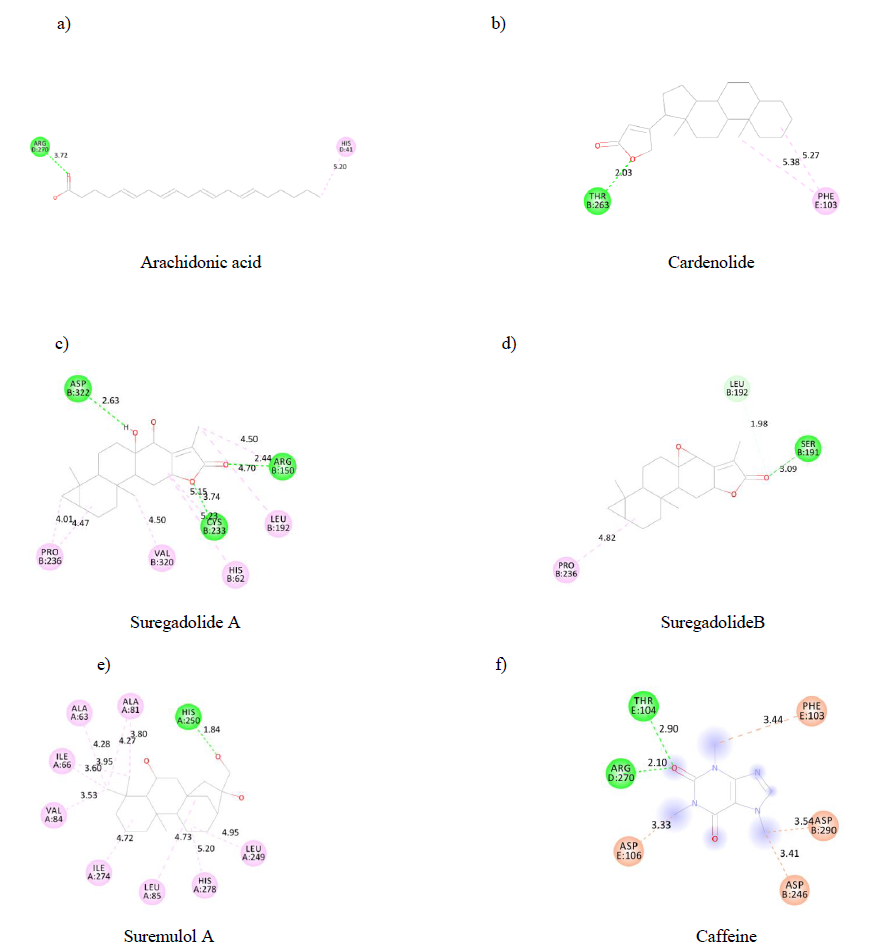
Figure3: 2D structure of substances binding sites on A2AR.
To determine druggability of the molecules, we analyzed the structure of the A2AR to distinguish drug and non-drug regions using the cavityplus online server. Results showed that A2AR consists of 33 cavities, of which four are druggable, five are less druggable, and the rest are undruggable. A set of aminoacid residues determines each cavity (supplementary table 2). Determining binding sites for each single molecule allowed us to determine the cavity where each natural molecule was attached (table 5). Results showed that suremulol A bound to cavity 6, which is druggable and has the strongest drugScore of 3364.00. Arachidonic acid bound to cavity1, and was druggable with a drugScore of 755.00. However, suregadolideA and suregadolideB bound to cavity 3, which is less druggable with a drugScoreof 174.00. However, cardenolide bound to cavity 16, which is undruggable presenting a drugScore of -602.00. And, caffein bound to cavity 3 and cavity 8 which were less druggable with a drugScore of 174.00 and -164.00 respectively. Our results revealed that suremulolA and suregadolideA are the best potential antagonists of A2AR.
Natural molecules |
Cavity No. |
Drugscore |
Druggablility |
Arachidonic acid |
1 |
755.00 |
Druggable |
2 |
28.00 |
lessdruggable |
|
Suregadolide A ; Suregadolide B; Caffein |
3 |
174.00 |
lessdruggable |
4 |
768.00 |
Druggable |
|
5 |
-2.00 |
lessdruggable |
|
Suremulol A |
6 |
3364.00 |
Druggable |
7 |
3310.00 |
Druggable |
|
Caffein |
8 |
-164.00 |
lessdruggable |
9 |
-320.00 |
Undruggable |
|
10 |
-288.00 |
Undruggable |
|
11 |
-672.00 |
Undruggable |
|
12 |
-768.00 |
Undruggable |
|
13 |
-215.00 |
Undruggable |
|
14 |
-783.00 |
Undruggable |
|
15 |
412.00 |
lessdruggable |
|
Cardenolide |
16 |
-602.00 |
Undruggable |
17 |
-247.00 |
Undruggable |
|
18 |
-814.00 |
Undruggable |
|
19 |
-775.00 |
Undruggable |
|
20 |
-1117.00 |
Undruggable |
|
21 |
-629.00 |
Undruggable |
|
22 |
-736.00 |
Undruggable |
|
23 |
-1078.00 |
Undruggable |
|
24 |
-883.00 |
Undruggable |
|
25 |
-600.00 |
Undruggable |
|
26 |
-988.00 |
Undruggable |
|
27 |
-1189.00 |
Undruggable |
|
28 |
-1222.00 |
Undruggable |
|
29 |
-922.00 |
Undruggable |
|
30 |
-816.00 |
Undruggable |
|
31 |
-1233.00 |
Undruggable |
|
32 |
-1337.00 |
Undruggable |
|
33 |
-1655.00 |
Undruggable |
Table 5: Druggability study of natural molecules-A2AR binding sites
Druglikeness of suremulolA and suregadolideA:
To define the druglikeness of our molecules (druglikeness used for fast calculation of drug-like properties of a molecule and identify the potential of a drug candidate for good absorption), we used the lipinski and veber rules. Results demonstrated that suremulolA and suregadolideA complied with both rules (table6). However, these had less than five hydrogen bound donors (HBD), less than 10 hydrogen bound acceptors (HBA), a molecular weight of less than 500 Da, and a partition coefficient log P of less than 5. Additionally, these exhibited a number of rotatable bounds (NBR) less than 10 and a polar surface area (PSA) of less than 140Å.
Lipinski rules |
Veber rules |
|||||
HBD<5 |
HBA<10 |
clogP<5 |
MW<500(Da) |
NBR<10 |
TPSA<140(Å) |
|
Suremulol A |
3 |
3 |
3.11 |
322.49 |
1 |
60.69 |
Suregadolide A |
2 |
4 |
2.58 |
332.44 |
0 |
66.76 |
Table 6: Druglikness prediction of Suremulol A and Suregadolide A
4. Discussion
Several studies have been eager to design new immunotherapeutic treatments against cancers, by targeting inhibitory immune checkpoint molecules, such as the A2AR, in order to boost the anti-tumor immune response [6]. Recently, many researchers have been interested in the use of natural molecules in order to avoid side effects that can occur by using antibody-based immunotherapy. Another objective is to make the therapy more accessible to the majority of patients by avoiding high costs [7,8]. The aim of our study was to search for adequate natural molecules that can be used as antagonists of the A2AR and that are in line with drug safety standards. Our study demonstrated that: 1) EnglerinA, eicosapentanoic acid and 6-acetoxy-anopterine downregulated A2AR expression, 2) SuremulolA, arachidonic acid, cardenolide, suregadolideB and suregadolideA exhibited suitable ADMET properties, 3) SuremulolA and suregadolideA presented high affinity and an important drug score, suggesting thatthese molecules could be suitable candidates for A2AR blockade. The first step consisted of choosing candidate antagonists capable of decreasing the expression of the A2AR. EnglerinA was found to be the only compound able to significantly inhibit the A2AR gene expression. Also, A2AR expression tended to decrease upon treatment with eicosapentanoic acid and 6-acetoxy-anopterine. Studies have demonstrated that, englerinA, a sesquiterpene isolated from the Tanzanian plant Phyllanthus engleri, was found to be a potent and selective inhibitor of renal cancer growth cell lines [16]. In addition, englerinA was more potent in several cases than the current standards of care, sunitinib, and sorafenib [17]. Our results exhibited the ability of these molecules to act on their specific targets. However, an effective drug must also have other characteristics. One of the significant challenges in drug development is the careful evaluation of human drug toxicity. Furthermore, several marketed drugs were withdrawn from the market due to serious adverse effects [15]. Toxicity profile prediction showed that englerinA induced inhibition of the Human either-a-go-go-related gene (hERG) which implicate in regulating cardiac excitability and stability of normal cardiac rhythm [16]. Additionally, the evaluation of hERG inhibition became a necessary pre-clinical assessment for candidate drugs [17]. Mutations in hERG may induce congenital long QT syndrome that has resulted in many drugs being removed from the market or terminated during clinical trials [18]. This led us to investigate other molecules similar to englerinA. We then assessed, using the fingerprint method, molecules with a similar structure to that of eicosapentanoic acid 6-acetoxy-anopterine. This method indeed allowed the identification of molecules with increased chance of displaying similar biological activities against the same target [19]. The first screening of eighty eight natural molecules was based on their toxicity profile. In our study, from 88 selected molecules, only 36 passed this selection process. Toxicity studies are crucial to determine possible mutagenic, carcinogenic, genotoxic, and heart health side effects. Mutagenicity is one of the most crucial endpoints of toxicity [20]. Additionally, the detection of mutagenicity upon drug discovery could stop the development of potentially dangerous drugs [21]. Equally, a genotoxic substance may promote carcinogenesis or laying the foundation for congenital disorders [22,23]. Another important criterion is hepatotoxicity. Liver toxicity still ranks among the highest reasons for drug attrition. Indeed, drug-induced liver injury is of great concern for patient safety [24]. Hepatic adverse effects in clinical trials often lead to late and costly termination of drug development systems. Thus, the early identification of a hepatotoxic potential is of great importance [24]. Indeed, once a drug molecule enters the body, it encounters various obstacles on its way, related to absorption, distribution, metabolism, and excretion, which are often referred to as ADME properties [10,14]. Once the non-toxic molecules have been selected, we performed absorption analysis, oral bioavailability and intestinal absorption. Our results showed that eight molecules present adequate absorption properties. Previous studies demonstrated that oral delivery is that the most accepted drug administration route among the varied delivery pathways [25]. However, in many cases, most orally administered drugs are metabolized and eliminated before reaching systemic blood circulation [26]. Therefore, poor bioavailability may cause a new drug to fail clinical trials, even if it had exhibited high efficacy in previous in vitro and in vivo tests [26]. Likewise, the prediction of human intestinal absorption (HIA) is crucial in developing oral drugs [27]. In our study, we found that only arachidonic acid, cardenolide, suregadolideA, suregadolideB, and suremulolA showed a good distribution capacity. During all the stages of the analysis procedure, we took into account the bioselectivity of the blood-brain barrier (BBB), which limits the diffusion of drugs. Almost 98% of all small molecules do not cross the BBB [28]. Another element limiting the distribution of drugs is P-glycoprotein (Pgp) that is expressed naturally on the plasmatic membranes of endothelial cells at the BBB [29], which plays a role in the multidrug resistance phenomenon, that leads to chemotherapy failure in cancer treatments [30]. Also, plasma protein binding (PPB) is an essential pharmacokinetic property of drug discovery molecules. Only the free form of the drug is pharmacologically active. However, The plasma protein-bound form is pharmacologically inactive and cannot diffuse to reach its target [31]. PPB is strongly associated with drug distribution, metabolism, and clearance, influencing drugs' efficacy and potency [31]. Furthermore, some studies consider the degree of drug binding to plasma proteins, high (85-98%) and very high (>98%) protein binding, whereas medium to low (<85%) protein binding [32]. Additionally, other studies consider that drugs with values higher than 0.9 are said to be highly bound, and those with values lower than 0.2 are said to show little or no plasma protein binding [33]. A final obstacle for any molecule is the metabolism (metabolism is carried out by enzymes such as cytochrome P450, contributing to about 75% of marketed drugs' metabolism), which poses a considerable threat to a drug's effectiveness. These enzymes are necessary for determining the pharmacokinetic profile of a drug [34]. Our results showed that suremulolA is observed to be a substrate for two cytochromes (CYP) enzymes, CYP3A4 and CYP2C9, and does not induce CYP inhibition. Furthermore, the other four ligands are the substrates for a single CYP enzyme. A compound that is a substrate or non-inhibitor of CYP450 means that it will not hamper the biotransformation of drugs metabolized by the CYP450 enzyme. Additionally, binding energy is the primary parameter that is generated as a result of molecular docking. It values the interaction strength and affinity ofthe molecules towards the A2AR. However, the affinity results in an energy score of the bond between the two molecules. The lower the energy score, the stronger the affinity. All selected molecules showed an energy score inferior to that of control, «caffein». Indeed, these low molecules binding energy score results in a strong affinity between candidate molecules and A2AR. Molecule binding sites are one of the major biochemical functions of proteins. Thus, the identification of binding sites is an interesting point for the A2AR interaction function. Our results indicate that cardenolide, suremulolA, arachidonic acid, and suregadolideB have one conventional hydrogen bond interaction with amino acids, caffein has two conventional hydrogen bonds and suregadolideA has three conventional hydrogen bonds. On the other hand, we observed other essential interactions such as hydrophobic, Pi-alkyl and alkyl. The hydrophobic interactions and conventional hydrogen bonding have essential contributions to the stabilization of the binding structures. Also, a strong inhibitor binding is reflected by the frequency of conventional hydrogen bonds [11,35]. Computational detection of protein cavities has been considered a vital step for structure-based drug design. The druggability value reflects the possibility of a cavity being a good target for binding drug-like molecules. Equally, CavityDrugScore was able to separate druggable and undruggable proteins successfully [36]. Results showed that suremulolA bound to the most druggable cavity with the strongest drugscore, followed by arachidonic acid, which boundtoa druggable cavity. Then, suregadolideA, suregadolideB and caffein that boundto a less druggable cavity with a moderate drug score. In contrast, the cardenolide bound to an undruggable cavity, suggesting that the cardenolide could not exert any therapeutic function during its interaction with A2AR. Moreover, affinity analysis and druggability results, revealed that suremulolA and suregadolideA were good potential antagonist candidates for A2AR. Besides, there are no violation of lipinski and veber rules for these two molecules.
5. Conclusion
Over the years, the process and criteria for accepting new drugs have become increasingly rigorous. Given increased costs and durations, in silico studies have become essential at an early stage of drug development. This helps in minimizing the cost of pre-clinical and clinical trials and thus minimize the risk of drug rejection. In our study, suremulol A and suregadolide A were selected as potential A2AR antagonist candidates. These two molecules exhibited properties, which were consistent with ADMET standards, lipinski and veber rules, and displayed a high drug score and a strong affinity to A2AR, supported by hydrogen and hydrophobic bonds. If confirmed, blocking A2AR in a cell-based approach, using suremulol A and/or suregadolide A, would lead to boosting the anti-tumor immune response.
6. Competing Interest
The authors declare no conflicts of interests
7. Funding
The Moroccan Ministry of Higher Education and Research (grant PPR1, type B for A.B.)
The Moroccan Ministry of Industry, Commerce, Green and Digital Economy. Digital development agency (IA and its applications for A.B.).
References
- Topalian SL, Drake CG, Pardoll DM. Immune Checkpoint Blockade: A common denominator approach to cancer therapy. Cancer Cell 27 (2015): 450-461.
- Ma SR, Deng WW, Liu JF et al. Blockade of Adenosine A2A receptor enhances CD8+ T cells response and decreases regulatory T Cells in Head and Neck Squamous Cell Carcinoma. Mol Cancer 16 (2017).
- Ohta A, Gorelik E, Prasad SJ,et al. A2A Adenosine receptor protects tumors from antitumor T Cells. Proc Natl AcadSci USA 103 (2006): 13132-13137.
- Sorrentino C, Hossain F, Rodriguez PC,et al. Adenosine A2A receptor stimulation inhibits TCR-induced Notch1 activation in CD8+T-Cells. Front Immunol 10 (2019).
- Ott M, Tomaszowski KH, Marisetty A,et al. Profiling of patients with glioma reveals the dominant immunosuppressive axis is refractory to immune function restoration. JCI Insight5 (2017).
- Kjaergaard J, Hatfield S, Jones G,et al. A2A adenosine receptor gene deletion or synthetic A2A antagonist liberate tumor-reactive CD8+ T Cells from Tumor-Induced Immunosuppression. J Immunol201 (2018): 782-791.
- Rawangkan A, Wongsirisin P, Namiki K,et al. Green tea catechin is an alternative immune checkpoint inhibitor that inhibits PD-L1 expression and lung tumor growth. Molecules23 (2018).
- Eini H, Frishman V, Yulzari R,et al. Caffeine promotes anti-tumor immune response during tumor initiation: Involvement of the Adenosine A2A Receptor. BiochemPharmacol98 (2015): 110-118.
- Bruno A, Costantino G, Sartori L,et al. The in silico drug discovery toolbox: Applications in lead discovery and optimization. Curr Med Chem26 (2019): 3838-3873.
- van de Waterbeemd H, Gifford E. ADMET in Silico Modelling: Towards Prediction Paradise? Nat Rev Drug Discov 2 (2003): 192-204.
- Arthur DE, Uzairu A. Molecular docking studies on the interaction of NCI anticancer analogues with human Phosphatidylinositol 4,5-Bisphosphate 3-Kinase catalytic subunit. Journal of King Saud University- Science 31 (2019): 1151-1166.
- Martín-Hernández R, Reglero G, Ordovás JM,et al. NutriGenomeDB: A Nutrigenomics Exploratory and Analytical Platform. Database 20 (2019).
- Zeng X, Zhang P, He W,et al. Natural product activity and species source database for natural product research, discovery and tool development. Nucleic Acids Research 46 (2018): D1217-D1222.
- Yang H, Lou C, Sun L,et al. AdmetSAR 2.0: Web-Service for Prediction and Optimization of Chemical ADMET Properties. Bioinformatics 35 (2019): 1067-1069.
- Xu Y, Wang S, Hu Q,et al. CavityPlus: A web server for protein cavity detection with Pharmacophore Modelling, allosteric site identification and covalent ligand binding ability prediction. Nucleic Acids Res 46 (2018): W374-W379.
- Wu Z, Zhao S, Fash DM,et al. Beutler JA. Englerins: A Comprehensive Review. J Nat Prod 80 (2017): 771-781.
- Li Z, Nakashige M, Chain W. A Brief Synthesis of (-)-Englerin A. Journal of the American Chemical Society133 (2011): 6553-6556.
- Li AP. Accurate prediction of human drug toxicity: A major challenge in drug development. Chemico-Biological Interactions 150 (2004): 3-7.
- Perrin MJ, Subbiah RN, Vandenberg JI,et al. Human Ether-a-Go-Go Related Gene (HERG) K+ Channels: Function and Dysfunction. Progress in Biophysics and Molecular Biology 98 (2008): 137-148.
- Zhou P, Babcock J, Liu L,et al. Activation of Human Ether-a-Go-Go Related Gene (HERG) Potassium Channels by Small Molecules. ActaPharmacol Sin 32 (2011): 781-788.
- Muegge I, Mukherjee P. An overview of molecular fingerprint similarity search in virtual screening. Expert opinion on drug discovery 11 (2016): 137-148.
- Xu C, Cheng F, Chen L,et al. In Silico prediction of chemical ames mutagenicity. J ChemInf Model 52 (2012): 2840-2847.
- Hsu KH, Su BH, Tu YS,et al. Mutagenicity in a Molecule: Identification of core structural features of mutagenicity using a scaffold analysis. Plos One 11 (2016): e0148900.
- Ranganatha R, Chakravarthy S, Sukumaran S. High-throughput approaches for Genotoxicity testing in drug development: Recent Advances. IJHTS 6 (2016) 1-12.
- Kwon M, Leibowitz ML, Lee JH. Small but Mighty: The causes and consequences of micronucleus rupture. ExpMol Med 52 (2020): 1777-1786.
- Mulliner D, Schmidt F, Stolte M,et al. Computational models for human and animal hepatotoxicity with a global application scope. Chem Res Toxicol 29 (2016): 757-767.
- Lin CH, Chen CH, Lin ZC,et al. Recent advances in oral delivery of drugs and bioactive natural products using solid lipid nanoparticles as the carriers. Journal of Food and Drug Analysis 25 (2017): 219-234.
- Kim M,Sedykh A, Chakravarti SK,et al. Critical Evaluation of Human Oral Bioavailability for Pharmaceutical Drugs by Using Various Cheminformatics Approaches. Pharm Res 31 (2014): 1002-1014.
- Hubatsch I, Ragnarsson EGE, Artursson P. Determination of drug permeability and prediction of drug absorption in Caco-2 Monolayers. Nat Protoc 2 (2007): 2111-2119.
- Pardridge WM. The Blood-Brain Barrier: Bottleneck in brain drug development. NeuroRx 2 (2005): 3-14.
- Aryal M, Fischer K, Gentile C,et al. Effects on P-Glycoprotein expression after blood-brain barrier disruption using focused ultrasound and microbubbles. Plos One 12 (2017).
- Li D, Chen L, Li Y,et al. ADMET evaluation in drug discovery. 13. Development of in Silico prediction models for P-Glycoprotein Substrates. Mol Pharm 11 (2014): 716-726.
- Bohnert T, Gan LS. Plasma Protein Binding: From Discovery to Development. Journal of Pharmaceutical Sciences 102 (2013): 2953-2994.
- Buscher B, Laakso S, Mascher H,et al. Bioanalysis for plasma protein binding studies in drug discovery and drug development: Views and Recommendations of the European Bioanalysis Forum. Bioanalysis 6 (2014): 673-682.
- Sparreboom A, Loos WJ. Protein Binding of Anticancer Drugs. In Handbook of Anticancer Pharmacokinetics and Pharmacodynamics; Figg WD, McLeod HL, Eds. Cancer Drug Discovery and Development Humana Press: Totowa, NJ 2004, pp 169-188.
- Brändén G, Sjögren T, Schnecke V,et al. Structure-Based ligand design to overcome CYP Inhibition in Drug Discovery Projects. Drug Discovery Today 19 (2014): 905-911.
- Billones J, Yang C. TowardsAntituberculosis Drugs: Molecular Docking of curcumin and its analogues to PantothenateSynthetase. Philippine Journal of Science 141 (2013): 187-196.
Supplementary Information
Symbol |
Log2 FC |
AverageExpr |
p-value |
Adjusted p-value |
Experiment (GEO id, Cell type, Treatment, Concentration, Duration) |
ADORA2A |
1.1 |
10.9 |
0.005 |
0.087 |
GSE56496SW620 Rosemary 30ug 48h |
ADORA2A |
0.7 |
7.4 |
0.003 |
0.035 |
GSE55897MDA MB436 Indole3carbinol 24h |
ADORA2A |
0.6 |
0.6 |
0.039 |
0.876 |
GSE44290PaCa2 Aaspera leaves 48h |
ADORA2A |
0.6 |
5.7 |
0.004 |
0.252 |
GSE50945Placental cells Fish oil |
ADORA2A |
0.5 |
6.9 |
0.009 |
0.335 |
GSE21976Caco2 Bifidobacterium bifidum 4h |
ADORA2A |
0.3 |
7.6 |
0.031 |
0.343 |
GSE10022422Rv1 Wchinensis Extract 16h |
ADORA2A |
0.2 |
6.1 |
0.028 |
0.59 |
GSE48668HeLa delta Tocotrienol 30min |
ADORA2A |
-0.3 |
2.9 |
0.085 |
1 |
GSE18589Myotubes Eicosapentaenoic acid 24h |
ADORA2A |
-0.3 |
6.7 |
0.004 |
0.124 |
GSE81277LNCaP 6AA 10nM 24h |
ADORA2A |
-1 |
0 |
0.001 |
0.042 |
GSE86046A498 EnglerinA 20h |
Supplementary table1: Technical and statistical data relating to the expression of A2AR gene in each experiment