Determinants of Preterm Births at a National Hospital in Zambia: Application of Partial Proportional Odds Model
Article Information
Moses Mukosha1, 2, 5*, Choolwe Jacobs2, Patrick Musonda2, John Mathias Zulu2, Sheila Masaku2, Chipo Nkwemu2, Bellington Vwalika3, Kunda Mutesu Kapembwa4, Patrick Kaonga2
1Department of Pharmacy, School of Health Sciences, University of Zambia, Lusaka, Zambia
2Department of Epidemiology & Biostatistics, School of Public Health, University of Zambia, Lusaka, Zambia
3Department of Obstetrics and Gynecology, School of Medicine, University of Zambia, Lusaka, Zambia
4Department of Neonatal Intensive Care Unit, Women and Newborn Hospital, Lusaka, Zambia
5Faculty of Health Sciences, School of Public Health, The University of Witwatersrand, Johannesburg, South Africa
*Corresponding Author: Moses Mukosha, The University of Zambia, Department of Pharmacy, BOX 50101, Ridgeway campus, Lusaka, Zambia
Received: 27 March 2021; Accepted: 06 April 2021; Published: 11 June 2021
Citation:
Moses Mukosha, Choolwe Jacobs, Patrick Musonda, John Mathias Zulu, Sheila Masaku, Chipo Nkwemu, Bellington Vwalika, Kunda Mutesu Kapembwa, Patrick Kaonga. Determinants of Preterm Births at a National Hospital in Zambia: Application of Partial Proportional Odds Model. Obstetrics and Gynecology Research 4 (2021): 117-130.
Share at FacebookAbstract
Background: Preterm birth (PTB), the delivery of a baby before 37 completed weeks of gestation, is responsible for increased childhood morbidity and mortality globally. However, in most developing countries, the determinats of PTB are usually underestimated and content-specific. Therefore, we assessed the determinants of ordered preterm birth levels at the Women and Newborn Teaching Hospital, Lusaka, Zambia.
Methods: We reviewed admission and delivery registers from 1st January 2018 to 30th September 2019 for birth records. Preterm births were in four categories; term, moderate, late preterm and very preterm. We assessed the determinants of ordered levels of preterm birth using the partial proportional odds regression model. Data were analysed using Stata version 16, and statistical tests were done using 5% significance level and 95% confidence interval.
Results: The study included a total of 3243 case records of women with a median age of 26 years (IQR, 22-33), of whom 399 (12.3%) delivered very preterm infants, 914 (28.18%) delivered moderate-term infants, 957 (29.51%) delivered late-term infants and 973 (30%) delivered term infants. There were disparities across infants born to HIV uninfected and HIV infected women, with the latter being more likely to be on the lower levels of preterm birth. However, attending antenatal clinic and a unit increase in maternal age were more likely to be on the higher levels of preterm birth. Pre-eclampsia's effect was not constant across the binary logistic regression models but generally showed a reduced odds of being in higher preterm birth levels for women with the condition.
Conclusion: HIV infection and pre-eclampsia predict lower preterm birth levels while attending antenatal care (ANC), and increased maternal age is protective. Pregnant women presenting with pre-eclampsia and HIV infection should receive special consider
Keywords
Partial Proportional Odds Model, Women and Newborn Teaching Hospital, Preterm Birth, Zambia
Partial Proportional Odds Model articles Partial Proportional Odds Model Research articles Partial Proportional Odds Model review articles Partial Proportional Odds Model PubMed articles Partial Proportional Odds Model PubMed Central articles Partial Proportional Odds Model 2023 articles Partial Proportional Odds Model 2024 articles Partial Proportional Odds Model Scopus articles Partial Proportional Odds Model impact factor journals Partial Proportional Odds Model Scopus journals Partial Proportional Odds Model PubMed journals Partial Proportional Odds Model medical journals Partial Proportional Odds Model free journals Partial Proportional Odds Model best journals Partial Proportional Odds Model top journals Partial Proportional Odds Model free medical journals Partial Proportional Odds Model famous journals Partial Proportional Odds Model Google Scholar indexed journals Women articles Women Research articles Women review articles Women PubMed articles Women PubMed Central articles Women 2023 articles Women 2024 articles Women Scopus articles Women impact factor journals Women Scopus journals Women PubMed journals Women medical journals Women free journals Women best journals Women top journals Women free medical journals Women famous journals Women Google Scholar indexed journals Newborn articles Newborn Research articles Newborn review articles Newborn PubMed articles Newborn PubMed Central articles Newborn 2023 articles Newborn 2024 articles Newborn Scopus articles Newborn impact factor journals Newborn Scopus journals Newborn PubMed journals Newborn medical journals Newborn free journals Newborn best journals Newborn top journals Newborn free medical journals Newborn famous journals Newborn Google Scholar indexed journals Preterm Birth articles Preterm Birth Research articles Preterm Birth review articles Preterm Birth PubMed articles Preterm Birth PubMed Central articles Preterm Birth 2023 articles Preterm Birth 2024 articles Preterm Birth Scopus articles Preterm Birth impact factor journals Preterm Birth Scopus journals Preterm Birth PubMed journals Preterm Birth medical journals Preterm Birth free journals Preterm Birth best journals Preterm Birth top journals Preterm Birth free medical journals Preterm Birth famous journals Preterm Birth Google Scholar indexed journals abnormal placentation articles abnormal placentation Research articles abnormal placentation review articles abnormal placentation PubMed articles abnormal placentation PubMed Central articles abnormal placentation 2023 articles abnormal placentation 2024 articles abnormal placentation Scopus articles abnormal placentation impact factor journals abnormal placentation Scopus journals abnormal placentation PubMed journals abnormal placentation medical journals abnormal placentation free journals abnormal placentation best journals abnormal placentation top journals abnormal placentation free medical journals abnormal placentation famous journals abnormal placentation Google Scholar indexed journals micronutrients deficiencies articles micronutrients deficiencies Research articles micronutrients deficiencies review articles micronutrients deficiencies PubMed articles micronutrients deficiencies PubMed Central articles micronutrients deficiencies 2023 articles micronutrients deficiencies 2024 articles micronutrients deficiencies Scopus articles micronutrients deficiencies impact factor journals micronutrients deficiencies Scopus journals micronutrients deficiencies PubMed journals micronutrients deficiencies medical journals micronutrients deficiencies free journals micronutrients deficiencies best journals micronutrients deficiencies top journals micronutrients deficiencies free medical journals micronutrients deficiencies famous journals micronutrients deficiencies Google Scholar indexed journals abnormal placenta articles abnormal placenta Research articles abnormal placenta review articles abnormal placenta PubMed articles abnormal placenta PubMed Central articles abnormal placenta 2023 articles abnormal placenta 2024 articles abnormal placenta Scopus articles abnormal placenta impact factor journals abnormal placenta Scopus journals abnormal placenta PubMed journals abnormal placenta medical journals abnormal placenta free journals abnormal placenta best journals abnormal placenta top journals abnormal placenta free medical journals abnormal placenta famous journals abnormal placenta Google Scholar indexed journals fetal growth articles fetal growth Research articles fetal growth review articles fetal growth PubMed articles fetal growth PubMed Central articles fetal growth 2023 articles fetal growth 2024 articles fetal growth Scopus articles fetal growth impact factor journals fetal growth Scopus journals fetal growth PubMed journals fetal growth medical journals fetal growth free journals fetal growth best journals fetal growth top journals fetal growth free medical journals fetal growth famous journals fetal growth Google Scholar indexed journals HIV articles HIV Research articles HIV review articles HIV PubMed articles HIV PubMed Central articles HIV 2023 articles HIV 2024 articles HIV Scopus articles HIV impact factor journals HIV Scopus journals HIV PubMed journals HIV medical journals HIV free journals HIV best journals HIV top journals HIV free medical journals HIV famous journals HIV Google Scholar indexed journals maternal age articles maternal age Research articles maternal age review articles maternal age PubMed articles maternal age PubMed Central articles maternal age 2023 articles maternal age 2024 articles maternal age Scopus articles maternal age impact factor journals maternal age Scopus journals maternal age PubMed journals maternal age medical journals maternal age free journals maternal age best journals maternal age top journals maternal age free medical journals maternal age famous journals maternal age Google Scholar indexed journals
Article Details
1. Introduction
Preterm birth refers to delivering a baby before 37 completed weeks of gestation and disproportionately affects the low and middle-income countries (LMIC) [1, 2]. The global burden of preterm births and the associated complications remain very high and content-specific [3]. Globally, about 15 million preterm births occur every year and of those, at least a million die due to prematurity complications [4]. Zambia shares the disproportionate burden of preterm birth with approximately 77,600 preterm births each year compared to similar settings in sub-Saharan Africa [5]. Despite this high rate of preterm births, the determinants are underestimated and not adequately known. Preterm birth is associated with various risk factors, and the common ones are placental complications such as pre-eclampsia, UTIs [6], history of preterm birth, substance use, fetal growth restriction, micronutrients deficiencies such as zinc, selenium, and copper. Others are haemorrhage, abnormal placentation, oligohydramnios and multi-foetal gestation [7-12]. Placental complications account for 14% of preterm births with spontaneous preterm labour, accounting for 40% [13]. While related risk factors and outcomes of preterm births are well documented in the literature, these vary in severity and occurrence for different world regions and are content-specific, with sub-Saharan Africa bearing the highest-burden compared to the developed countries [14].
Other determinants of preterm birth are HIV infection, advanced maternal age and pregnancy-induced hypertension [15]. Several studies that have reported the factors associated with preterm birth have used logistic regression models to compare between term and preterm infants, potentially missing the within differences between the two categories. Dependent on how early a baby is born, a birth can be: term (i.e., more than 37 weeks gestation), moderate preterm (i.e., 32 to 34 weeks gestation), late preterm (i.e., 34 to 36 weeks gestation) very preterm (i.e., less than 32 weeks gestation) [16]. However, studies that have examined the determinants of preterm births, especially considering its ordinal nature in Zambia are lacking. Understanding the determinants of different content-specific levels of preterm birth is necessary to inform clinical management and/care, provide information to physicians on risk level stratification, and target and evaluate interventions. Therefore, this study aimed at assessing the determinants of ordered levels of preterm
births at a National Hospital in Lusaka, Zambia.
2. Methods
2.1 Study design and setting
We conducted a cross-sectional study at the Women and Newborn Teaching Hospital in Lusaka, Zambia. The hospital is the largest referral centre in Zambia for obstetric, gynaecological and neonatal conditions [17]. On average, 28,800 admissions of pregnant women per year and about 18,000 births per year are recorded at this hospital. The hospital receives referrals from over 20 clinics and five first-level hospitals from surrounding areas of Lusaka and other parts of the country.
2.2 Study population and sampling technique
This study population comprised women who delivered at Women and New Born Hospital between 1st January 2018 to 30th September 2019. We conducted a complete enumeration of eligible clinical records. To be considered for further review, we screened files for completeness of the information. Files found to have more than 10% missing information for required variables were excluded. The total sample of records was subdivided into four levels of preterm birth: term (≥ 37 weeks), very preterm (≤ 27 weeks), moderate preterm (28 weeks to 32 weeks), and late preterm (33 to < 37 weeks). After screening the files, de facto eligible sample came to 3243 records (Figure 1).
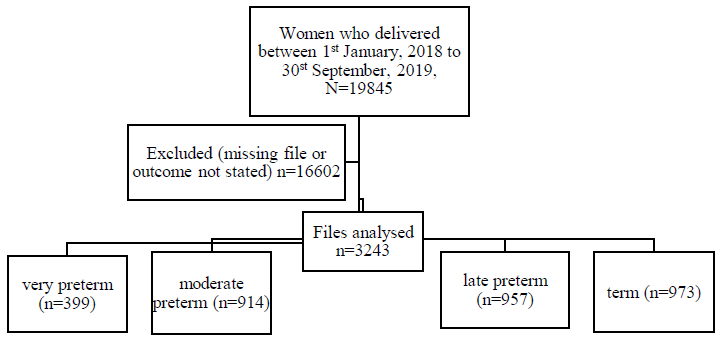
Figure 1: Flow chart of the sampling frame for the records.
2.3 Data extraction
The study extracted data from the admission and delivery records from 1st January 2018 to 30th September 2019. For data entry, we used a form of predesigned and Excel-based sheet. We audited all entered information at the end of each day to ensure its accuracy and completeness. Relevant demographic and medical data were extracted from the medical records. Demographic, obstetric and clinical data included antenatal care (ANC) attendance, maternal age, gestational age at delivery, maternal HIV status, gravidity, parity, sex of baby, history of preterm birth, pregnancy-induced hypertension and substance use. We entered the data into Microsoft excel operating system. We then carried out data cleaning, coding and validation until the database corresponded with the forms' data. After that, we exported the data to Stata/IC version 16 (Stata Corporation, College Station, Texas, USA) for
analysis.
2.4 Study outcome
We used preterm birth as an ordinal dependent variable, and it was defined as follows:
This study's independent variables were chosen based on literature [18-21]. These were maternal age (number of years); gravidity (number of pregnancies before); parity (number of living children); pre-eclampsia (yes, no); sex of infant (male, female); HIV status of the mother (positive, negative); employed (yes, no); ANC attendance (yes, no), history of preterm (yes, no) and haemorrhage (yes, no).
2.5 Statistical analysis
We used the median (interquartile range [IQR]) for continuous values (i.e. maternal age) after testing for the assumption of normality using the Shapiro-Wilk W-test and graphically Q-Q-plots. For the comparison of baseline characteristics across levels of preterm birth, we used the Kruskal Wallis test, and Fisher's exact test or Pearson chi-square test were applicable. We conducted data analysis in two stages to identify the potential determinates for levels of preterm birth. Firstly, we fitted the proportional odds model (POM), considering preterm's ordinal nature. After analysis, we observed that the test for POM assumption, done using a user-written command "brant" test, showed that one variable (pre-eclampsia) failed the model assumption as its p-value was significant (p=0.009), but the rest had insignificant p-values, which we further confirmed by parallel lines plot [22]. In the second analysis, before making a final decision, we used a suitable alternative model, the partial proportional ordinal model (PPOM). The main advantage of PPOM is that it relaxes the parallel lines assumption for explanatory variables across the outcome variable levels. In that regard, it is more accurate than the proportional ordinal regression model, and it has better parsimonious than the multinomial regression model. To fit the PPOM to the data, we used a user-written command "gologit2" [23] with a relaxed parallel regression assumption. According to R Williams [23], the general model of PPOM is written as equation one below:
Where M represents the number of levels of the ordinal preterm birth variable (i.e. 4). β is a vector of logit coefficients, X is a vector of independent variables, j is a cutpoint. We explored possible interactions between the significant factors. After that, the goodness-of-fit was assessed by the deviance test, the log-likelihood and the pseudo R2. The analysis used a significance level of 5% and 95% confidence intervals. We analysed the data with Stata/IC version 16 (Stata Corp., College Station, Texas, USA).
2.6 Ethical considerations
The University of Zambia Biomedical Research Ethics Committee (UNZABREC) approved this study; reference number UNZA-221/2019. Additional permission was obtained from Women and Newborn Hospital management to extract data and conduct the hospital study. This study used medical records from maternal files and no direct contact with participants; therefore, no informed consent was obtained. Data were de-identified to protect the participant's confidentiality.
3. Results
3.1 Clinical and demographic characteristics of study participants
The baseline characteristics of women by deliveries at Women and Newborn Hospital during the study period are shown in Table 1. A total of 3243 case records of women with a median age of 26 years (IQR, 22-33) were included in the study. From the total delivered, 399 (12.3%) were very preterm infants, 914 (28.2%) were moderate preterm, 957 (29.5%) were late preterm, and 973 (30.0%) were term infants. From the total number of women, 251 (7.7%) had pre-eclampsia, 38 (1.2%) experienced haemorrhage, and 2696 (83.1%) attended ANC during pregnancy, 18 (0.6%) had a history of pre-eclampsia, and 176 (5.4%) were in employment. From the univariate analysis, participants maternal age (median: very preterm [25 years] vs moderate preterm [26 years] vs late preterm [26 years] vs term [27 years]; p<0.001) was significantly different across the different categories of preterm birth. Additionally, there was evidence of a difference in parity (p=0.003), HIV status (p=0.002), sex of the baby (p<0.001), ANC attendance (p=0.004) and gravidity (p=0.007) across all categories of preterm birth. We did not find any association between preterm birth categories and employment status (p=0.528) and preterm birth history (p=0.971).
3.2 Proportional odds model (POM)
The overall score test for the proportional odds assumption: Chi-square = 16.39, df=10, p-value =0.089, In this case, not statistically significant, providing evidence that the parallel regression assumption for the overall model is not violated. However, the parallel regression assumption was violated for pre-eclampsia (X2(2) =9.37, p=0.009 for the score test), indicating odds ratio heterogeneity in the compared categories. Since pre-eclampsia violates the proportional odds assumption, its effect was allowed to vary across the
three binary models using the PPOM (Table 2).
3.3 Partial proportional odds model (PPOM)
In the final regression model, we conducted a PPOM comprising of three equations. In the first equation, the odds ratios of very preterm were compared to a combined group of moderate-term, late preterm and term. In the second equation, a combination of the very preterm and moderate-term were compared to a combined late preterm and term group. In the third equation, very preterm, moderate and late preterm were compared to term. The results of a PPOM are shown in Table 2. The log-likelihood ratio chi-square test LRX2(7)=79.29, p<0.001 for this model indicates that the full model shows a better fit than the null model with no independent variables in predicting the levels of gestational age. The Wald test of parallel lines assumption for the final model: chi-square test = 6.82, df=8, p=0.5561 indicates that the final model does not violet the proportional odds assumption.
This model indicates that the factors associated with preterm birth levels among the women were a unit increase in maternal age (years), sex of the baby (i.e. male compared to females), HIV infection, ANC attendance and pre-eclampsia. Compared to female infants, male infants (aOR= 1.46, 95% CI: 1.26-1.68, p<0.001), attending antenatal clinic (aOR= 2.06, 95% CI:1.28-3.30, p=0.003) and a unit increase in maternal age (aOR= 1.02, 95% CI: 1.01-1.03, p< 0.001) were associated with higher levels of preterm birth controlling for HIV status and preeclampsia. The infants born from HIV infected women were more likely to be in lower preterm birth levels than their counterparts born from HIV negative mothers (aOR= 0.67, 95% CI: 0.58-0.81, p<0.001). Overall, pre-eclampsia was associated with a 25% and 38% reduction in the likelihood of an infant being in higher levels of gestational age when we compared very preterm & moderate-term vs (late preterm & term) infants (95% CI: 0.56-1.01, p<0.059) and very preterm, moderate & late preterm vs (term) infants (95% CI: 0.44-0.87, p=0.006) binary logistic regression models respectively.
Characteristic |
Levels of preterm birth |
P-value |
|||
V. preterm n=399 (12.3%) |
M. preterm n=914 (28.2%) |
L. preterm n=957 (29.5%) |
Term n=973 (30%) |
||
Maternal age (IQR) |
25 (20, 31) |
26 (22, 32) |
26 (21, 33) |
27 (22-33) |
<0.001a |
Gravidity (IQR) |
2 (1, 3) |
2 (1, 4) |
2 (1, 4) |
2 (1, 4) |
0.007a |
Parity (IQR) |
2 (1, 3) |
2 (1, 4) |
2 (1, 4) |
2 (1, 4) |
<0.001a |
Employed |
|||||
Yes |
16 (9.25) |
52 (30.06) |
50 (28.9) |
55 (31.79) |
|
No |
308 (12.78) |
683 (28.34) |
718 (29.79) |
701 (29.09) |
0.528b |
Pre-eclampsia |
|||||
Yes |
23 (9.31) |
90 (36.44) |
73 (29.55) |
61 (24.70) |
|
No |
373 (12.55) |
816 (27.47) |
878 (29.55) |
904 (30.43) |
0.012b |
HIV status |
|||||
Positive |
68 (11.89) |
197 (34.44) |
164 (28.67) |
143 (25) |
|
Negative |
309 (11.92) |
707 (27.27) |
769 (29.66) |
808 (31.16) |
0.002 |
Sex of infant |
|||||
Female |
207 (14.33) |
448 (31) |
418 (28.93) |
372 (25.74) |
|
Male |
189 (10.55) |
466 (26.02) |
122 (44.7) |
599 (33.45) |
<0.001 |
ANC attendance |
|||||
Yes |
321 (12.18) |
751 (28.49) |
781 (29.63) |
783 (29.70) |
|
No |
17 (26.56) |
16 (25) |
19 (29.69) |
12 (18.75) |
0.004b |
History of preterm birth |
|||||
Yes |
2 (11.76) |
4 (23.53) |
6 (35.29) |
5 (29.41) |
|
No |
395 (12.3) |
905 (28.18) |
949 (29.55) |
963 (29.98) |
0.971c |
Haemorrhage |
|||||
Yes |
1 (2.63) |
12 (31.58) |
12 (31.58) |
13 (34.21) |
|
No |
396 (12.4) |
903 (28.25) |
939 (29.41) |
956 (29.94) |
0.289c |
IQR-Interquartile range, ANC-Antenatal Care, N-population, n-sample size, a=Kruskal Wallis test, b= Pearson chi-square test, c= Fisher's exact test, values are percentages and frequency, V-very, M-moderate, L-late
Table 1: Baseline characteristics of women by deliveries at Women and Newborn Hospital, Lusaka, Zambia (N=3243).
Variable |
Comparison |
||||||||
Very preterm vs. (moderate term, late preterm & term) |
Very preterm & moderate-term vs. (late preterm & term) |
Very preterm, moderate & late preterm vs. ( term) |
|||||||
OR1 |
CI |
P-value |
OR2 |
CI |
P-value |
OR3 |
CI |
P-value |
|
Maternal age (years) |
1.02 |
1.01, 1.03 |
<0.001 |
1.02 |
1.01, 1.03 |
<0.001 |
1.02 |
1.01, 1.03 |
<0.001 |
Pre-eclampsia |
1.41 |
0.86, 2.34 |
0.176 |
0.75 |
0.56, 1.01 |
0.059 |
0.62 |
0.44, 0.87 |
0.006 |
HIVstatus |
|||||||||
Negative |
ref |
ref |
ref |
||||||
Positive |
0.67 |
0.56, 0.81 |
<0.001 |
0.67 |
0.56, 0.81 |
<0.001 |
0.67 |
0.56, 0.81 |
<0.001 |
Infant |
|||||||||
Female |
ref |
ref |
ref |
||||||
Male |
1.46 |
1.26, 1.68 |
<0.001 |
1.46 |
1.26, 1.68 |
<0.001 |
1.46 |
1.26, 1.68 |
<0.001 |
ANC |
2.06 |
1.28, 3.30 |
0.003 |
2.06 |
1.28, 3.30 |
0.003 |
2.06 |
1.28, 3.30 |
0.003 |
The parallel lines assumption for the final model Wald test: chi2 = 6.82, df=8, P=0.5561, Test of overall model goodness-of-fit test (Likelihood Ratio): Chi-square = 79.29, df = 7, p-value < 0.001, Pseudo R2 = 0.018 |
Key: OR-odds ratio; CI-confidence intervals; ANC-antenatal care attendance; Ref= reference category
Table 2: Results of multiple PPOM using preterm birth as an outcome with four ordered categories.
4. Discussion
This study set out to explore determinats of levels of preterm births among women who delivered at Women and Newborn Hospital in Lusaka, Zambia. We found that slightly over one in every ten births were very preterm infants compared to three in every ten births for moderate and late preterm infants. Additionally, we found that the factors associated with lower preterm birth levels (i.e. very preterm birth) were HIV infection and pre-eclampsia. On the other hand, a unit increase in maternal age, sex of the baby (i.e. male compared to females) and attending ANC increased the odds of a higher category of preterm birth. Consistent with the extant literature [24-27], positive HIV serostatus has previously been linked to preterm birth and increased risk of neonates' mortality. A systematic review and meta-analysis of perinatal outcomes associated with maternal HIV infection reported higher odds of preterm delivery among HIV infected women [28]. Similarly, studies in Ethiopia and Kenya with a setting similar to Zambia reported a higher rate of preterm deliveries among HIV infected women [29, 30]. The underlying mechanism of how HIV is associated with preterm birth is still poorly understood. Studies have reported various pathways, and prominent among them is that HIV infection is characterised by high levels of abnormal inflammatory biomarkers such as C-reactive protein (hs-CRP), interleukin (IL)-6, IL-8, tumour necrosis factor-alpha (TNF-α), which have previously been linked to high preterm birth deliveries [28, 31-33].
Additionally, HIV infection has been linked to increased overexpression of the Type 1 T helper (Th cytokines, which are thought to be responsible for adverse pregnancy outcomes [34]. All women who are HIV positive in our study setting receive antiretroviral therapy (ART) as a routine standard of care. Our study, however, was not able to quantify time on ART which could have given us the required adjustments for the observed results. Furthermore, we did not have adherence data, although a pilot study which we recently conducted in a similar setting showed 96% adherence levels among pregnant women [35]. However, in contrast to the extant literature that mainly compares term and preterm infant, our study provides insight into the consistent effect of HIV across the levels of preterm birth. The observed differences between preterm birth categories are hypothesis-generating and could offer new preterm birth risk stratification opportunities.
In our study, pre-eclampsia was associated with higher odds of very preterm delivery. The effect of pre-eclampsia was, however, not consistent across all the levels of gestational age. Our study's findings on pre-eclampsia are in agreement with other similar studies [18, 20]. For instance, a large population-based cohort study found that women with pre-eclampsia were almost four and half times more likely to deliver preterm babies compared to their counterparts without pre-eclampsia [29, 30]. Pre-eclampsia's role in preterm delivery is thought to be through the inflammatory intermediates that characterise this condition [36, 37]. Recently, Ross et al. [36] studied women who delivered preterm infants and found that those who developed pre-eclampsia during pregnancy had different metabolic markers. The findings were suggestive of biological plausibility for pre-eclampsia to be a precursor to preterm birth. The other possible explanation of the link could be that pre-eclampsia in many instances, leads to the infant's therapeutic delivery as the only way to serve the mother and the infant when symptomatic management fails. Therefore, one would expect a woman who develops pre-eclampsia to have a higher chance of early delivery before term when complications arise.
Moreover, pregnancy-induced hypertension complica-tions, such as pre-eclampsia, can cause vascular damage to the placenta, hence inducing the oxytocin receptors, which results in preterm labour and delivery [38]. In contrast to similar studies, our findings demonstrated that the effect of pre-eclampsia is not consistent for all levels of preterm birth. The effect was higher for lower preterm birth levels, which could partly explain the high adverse maternal outcomes associated with pre-eclampsia [39, 40]. This finding is critical to public health efforts to improve preeclamptic women's overall health through increased monitoring and follow-up to reduce adverse maternal outcomes in this high-risk group. We found a positive association between maternal age and gestational age. For a unit increase in maternal age, a woman was more likely to have a term birth. However, there is currently no consensus on maternal age's effects on preterm birth in the extant literature [41-45]. One plausible explanation for the inconsistencies could be that younger mother's physiologic immaturity predisposes them to a higher chance of delivering a preterm infant. On the other hand, the prevalence of the preexisting chronic disease is more significant among older mothers and could be a risk factor for preterm birth [45]. The observed heterogeneity of risk for preterm births based on maternal age suggests the need for varying preterm birth prevention strategies across the reproductive life span. A multicentre study in Brazil on spontaneous preterm birth (SPB) found that maternal age and previous preterm delivery, among other clinical characteristics, predicted higher chances of preterm birth [46].
A systematic review of preterm birth risks for infants born to two groups of young mothers suggested a plausible reason for the observed association between preterm birth and maternal age due to biological changes or residual confounding [47]. It was not surprising to find that antenatal care (ANC) attendance was associated with an increased odds of a higher category of preterm birth by 2-fold [45]. ANC, in many aspects, is thought to be an effective method of improving pregnancy outcomes [48]. A study done in Kenya showed that mothers who had no ANC visit were 1.5 times more likely to deliver a preterm infant than their counterparts who attended ANC [49]. ANC offers an opportunity to monitor the pregnancy closely and educate the mothers on improving pregnancy outcomes [50]. Our study has implications for public health policy. ANC can offer important opportunities for linking the health system and the community, thereby improving pregnant women's overall health outcomes. Therefore, our findings support the interventions aimed at increasing ANC uptake to improve pregnancy outcomes. HIV infected women and those diagnosed with pre-eclampsia should be monitored closely during pregnancy as they are at a higher risk of preterm delivery. Programmes aimed at improving pregnancy outcomes in this high-risk population should be supported.
Our study has several strengths. First, the study used a large data set, giving us adequate power to detect differences in preterm birth categories compared to similar studies. Second, we conducted this study in a setting with a very high preterm birth rate and associated complications, enabling us to assess various known risk factors. Additionally, most previous studies used a logistic regression model, which cannot compare preterm birth levels. The PPOM elicits more information than the multinomial and binary logit regression techniques. Our study demonstrated that the effect of risk factors across the four categories of preterm birth might not be constant, and the partial proportional odds model, which allows such risk factors to vary across the binary logistic models, may explain the data well.
However, our study has some limitations worth noting. First, we were unable to measure time on ART since all HIV infected pregnant women are on ART which could have confounded the observed effect of HIV on preterm birth. Second, we could not control for some maternal variables like type of pregnancies (multiple or singleton gestation), diseases during pregnancy, and place of delivery as these were missing in the files. The potential endogeneity of the independent variables is another limitation of our study. Further, this study is based on programmatic data; hence, the study has all biases associated with observation study for which particular confounders cannot be controlled for from the study's onset as one would do in a clinical trial.
5. Conclusion
The odds of a lower level of preterm birth is higher if a mother has HIV infection and develop pre-eclampsia during pregnancy. On the other hand, a unit increase in maternal age and antenatal care attendance at least once reduced the odds of a lower level of preterm birth. Special consideration should be given to pregnant women with pre-eclampsia and HIV infection to improve pregnancy outcomes. Our findings support interventions aimed at increasing ANC uptake in the Zambian and other sub-Saharan Africa settings.
Abbreviations
CI: Confidence Interval; HIV: Human Immunodeficiency Virus; LMICs: low and middle-income countries; NCR: Neonatal Case Record; NICU: Neonatal Intensive Care Unit; PTB: Preterm Birth; UNZA: University of Zambia; UNZABREC: University of Zambia Biomedical Research Ethics Committee; WHO: World Health Organisation.
Acknowledgements
The authors would like to thank the members of staff at Women and Newborn Hospital. Special thanks also go to the management of Women and Newborn Hospital to permit us to use their data.
Funding
This was a self-funded study and did not receive any form of funding from anywhere.
Availability of Data and Materials
The data we used and support the findings of this study are available from the Women and Newborn Hospital, but restrictions apply to these data's availability, which were used with permission and not publicly available. Nevertheless, Data are available from the authors but only upon reasonable request and with permission of the Women and Newborn Hospital Lusaka Zambia.
Authors Contributions
MM, CJ, PK conceived the study, contributed to the study design, data collection, data analysis, data interpretation, and writing of the manuscript. BV, KMK contributed to the study design, data collection instruments and data interpretation. PM; data interpretation, data analysis and writing of the manuscript. JMZ, SM, CN contributed to data interpretation and writing of the manuscript. All authors read and approved the final draft of the manuscript.
Authors' Information
MM is a lecturer at the University of Zambia's School of Health Sciences in the Department of Pharmacy and an MSc student of Medical statistics, with the following qualifications: MSc, MSc. KMK is a Consultant Paediatrician & Neonatologist in the department of Neonatology Women and Newborn Hospital and a National Coordinator for neonatal services in the Ministry of Health Zambia with the following qualifications: MPhil, MMed, MBChB, BScHB, cert Neonatology. PM is a Professor and lead statistician at the University of Zambia's School of Public Health in the Department of Epidemiology and Biostatistics and has the following qualifications: Dip, BSc, MSc, PhD. BV is an associate Professor of Obstetrics and Gynaecology, University of Zambia School of Medicine and Head of training. BV is also a Research Professor, Global Women's Health, the University of North Carolina at Chapel Hill, Department of Obstetrics. CJ is an assistant registrar for postgraduate studies in the School of Public Health and a senior lecturer in the Department of Epidemiology and Biostatistics with the following qualifications: Dip, BSc, MPH, PhD. PK is a lecturer in the School of Public Health Department of Epidemiology and Biostatistics with the following qualifications: BSc, MPH, PhD. JMZ, CN and SM are medical statistics students in the Department of Epidemiology & Biostatistics School of Public Health, the University of Zambia.
Ethics Approval and Consent to Participate
This study was approved by the University of Zambia Biomedical Research Ethics Committee UNZABREC (ref: UNZA-221/2019). We did not get any written consent from participants as the study used secondary data and hence had no direct contact with participants. Nevertheless, we obtained permission from Women and Newborn Hospital management to extract data and conduct the study at the hospital.
Competing Interests
All the authors declare that they have no competing interests.
References
- Lee AC, Blencowe H, Lawn JE. Small babies,big numbers: global estimates of preterm birth. The Lancet Global Health 7 (2019): 2-3.
- Goldenberg RL, Muhe L, Saleem S, et al. Criteria for assigning cause of death for stillbirths and neonatal deaths in research studies in low-middle income countries. In.: Taylor & Francis (2019): 1915-1923.
- Ayebare E, Ntuyo P, Malande OO, et al. Maternal, reproductive and obstetric factors associated with preterm births in Mulago Hospital, Kampala, Uganda: a case control study. The Pan African medical journal 30 (2018): 272.
- Liu L, Oza S, Hogan D, et al. Global, regional, and national causes of under-5 mortality in 2000–15: an updated systematic analysis with implications for the Sustainable Development Goals. The Lancet 388 (2016): 3027-3035.
- Castillo MC, Fuseini NM, Rittenhouse K, et al. The Zambian Preterm Birth Prevention Study (ZAPPS): Cohort characteristics at enrollment. Gates open research (2018): 2-25.
- Mukosha M, Nambela L, Mwila C, et al. Urinary tract infections and associated factors in HIV infected pregnant women at a tertiary hospital in Lusaka, Zambia. The Pan African Medical Journal 37 (2020): 328.
- Chababa L, Mukosha M, Sijumbila G, et al. Relationship between serum zinc levels and pre-eclampsia at the University Teaching Hospital, Lusaka, Zambia. Medical Journal of Zambia 43 (2016): 139-144.
- Robinson JN, Norwitz E. Preterm birth: Risk factors, interventions for risk reduction, and maternal prognosis. Up to date (2021).
- Delnord M, Blondel B, Prunet C, et al. Are risk factors for preterm and early-term live singleton birth the same? A population-based study in France. BMJ open 8 (2018): 018745.
- Kwangu M, Yassa P, Lambwe N, et al. Interplay between nitric oxide (NO) and glucose 6-phosphate dehydrogenase (G6PD) activity in primary hypertension. International Journal of Medicine and Medical Sciences 6 (2014): 165-170.
- Chisulo A, Lambwe N, Sijumbila G, et al. Serum Selenium levels in Essential hypertension among adults at the University Teaching Hospital, Lusaka, Zambia. Medical Journal of Zambia 44 (2017): 218-224.
- Mukosha M. The prevalence of hyponatremia and effects of aminophylline on serum sodium ions in premature neonates admitted to Neonatal Intensive Care Unit, at the University Teaching Hospital, Lusaka, Zambia. Medicine (2015).
- Blencowe H, Cousens S, Chou D, et al. Born too soon: the global epidemiology of 15 million preterm births. Reproductive health 10 (2013): S2.
- Chawanpaiboon S, Vogel JP, Moller A-B, et al. Global, regional, and national estimates of levels of preterm birth in 2014: a systematic review and modelling analysis. The Lancet Global Health 7 (2019): 37-46.
- Gebreslasie K. Preterm birth and associated factors among mothers who gave birth in Gondar Town Health Institutions. Advances in Nursing (2016): 2016.
- Mitku AA, Zewotir T, North D, et al. Modeling Differential Effects of Maternal Dietary Patterns across Severity Levels of Preterm Birth Using a Partial Proportional Odds Model. Scientific reports 10 (2020): 1-10.
- Moses M. The prevalence of hyponatremia and effects of aminophylline on serum sodium ions in premature neonates admitted to Neonatal Intensive Care Unit, at the University Teaching Hospital, Lusaka, Zambia (2020).
- Laelago T, Yohannes T, Tsige G. Determinants of preterm birth among mothers who gave birth in East Africa: systematic review and meta-analysis. Italian Journal of Pediatrics 46 (2020): 10.
- Wassie M, Manaye Y, Abeje G, et al. Determinants of Preterm Birth among Newborns Delivered in Bahir Dar City Public Hospitals, North West Ethiopia (2020).
- Mekuriyaw AM, Mihret MS, Yismaw AE. Determinants of Preterm Birth among Women Who Gave Birth in Amhara Region Referral Hospitals, Northern Ethiopia, 2018: Institutional Based Case Control Study. International Journal of Pediatrics (2020).
- Rahman A, Rahman M, Pervin J, et al. Time trends and sociodemographic determinants of preterm births in pregnancy cohorts in Matlab, Bangladesh, 1990–2014. BMJ global health 4 (2019): 001462.
- Brant R. Assessing proportionality in the proportional odds model for ordinal logistic regression. Biometrics (1990): 1171-1178.
- Williams R. Generalized ordered logit/partial proportional odds models for ordinal dependent variables. The Stata Journal 6 (2006): 58-82.
- Slyker JA, Patterson J, Ambler G, et al. Correlates and outcomes of preterm birth, low birth weight, and small for gestational age in HIV-exposed uninfected infants. BMC pregnancy and childbirth 14 (2014): 7.
- Price JT, Phiri WM, Freeman BL, et al. Vaginal progesterone to prevent preterm delivery among HIV-infected pregnant women in Zambia: A feasibility study. Plos one 15 (2020): 0224874.
- Santosa WB, Staines-Urias E, Tshivuila-Matala CO, et al. Perinatal outcomes associated with maternal HIV and antiretroviral therapy in pregnancies with accurate gestational age in South Africa. Aids 33 (2019): 1623-1633.
- Yang M, Wang Y, Chen Y, et al. Impact of maternal HIV infection on pregnancy outcomes in southwestern China–a hospital registry based study. Epidemiology & Infection 147 (2019): 1-9.
- Wedi CO, Kirtley S, Hopewell S, et al. Perinatal outcomes associated with maternal HIV infection: a systematic review and meta-analysis. The lancet HIV 3 (2016): 33-48.
- Mekonen DG, Yismaw AE, Nigussie TS, et al. Proportion of Preterm birth and associated factors among mothers who gave birth in Debretabor town health institutions, northwest, Ethiopia. BMC research notes 12 (2019): 2.
- Obimbo MM, Zhou Y, McMaster MT, et al. Placental structure in preterm birth among HIV-positive versus HIV-negative women in Kenya. Journal of acquired immune deficiency syndromes (1999) 80 (2019): 94.
- Malaba TR, Phillips T, Le Roux S, et al. Antiretroviral therapy use during pregnancy and adverse birth outcomes in South African women. International journal of epidemiology 46 (2017): 1678-1689.
- Xiao P-L, Zhou Y-B, Chen Y, et al. Association between maternal HIV infection and low birth weight and prematurity: a meta-analysis of cohort studies. BMC pregnancy and childbirth 15 (2015): 246.
- Conroy AL, McDonald CR, Gamble JL, et al Altered angiogenesis as a common mechanism underlying preterm birth, small for gestational age, and stillbirth in women living with HIV. American journal of obstetrics and gynecology 217 (2017): 681-684.
- Maharaj NR, Phulukdaree A, Nagiah S, etal. Pro-inflammatory cytokine levels in HIV infected and uninfected pregnant women with and without pre-eclampsia. PloS one 12 (2017): 0170063.
- Mukosha M, Chiyesu G, Vwalika B. Adherence to antiretroviral therapy among HIV infected pregnant women in public health sectors: a pilot of Chilenje level one Hospital Lusaka, Zambia. The Pan African medical journal 35 (2020): 49-49.
- Ross KM, Baer RJ, Ryckman K, et al. Second trimester inflammatory and metabolic markers in women delivering preterm with and without pre-eclampsia. Journal of Perinatology 39 (2019): 314-320.
- Jelliffe-Pawlowski LL, Rand L, Bedell B, et al. Prediction of preterm birth with and without pre-eclampsia using mid-pregnancy immune and growth-related molecular factors and maternal characteristics. Journal of Perinatology 38 (2018): 963-972.
- Goldenberg RL, Culhane JF, Iams JD, et al. Epidemiology and causes of preterm birth. The lancet 371 (2008): 75-84.
- Mirkovic L, Tulic I, Stankovic S, et al. Prediction of adverse maternal outcomes of early severe pre-eclampsia. Pregnancy Hypertension 22 (2020): 144-150.
- Ndwiga C, Odwe G, Pooja S, et al. Warren C: Clinical presentation and outcomes of pre-eclampsia and eclampsia at a national hospital, Kenya: A retrospective cohort study. Plos one 15 (2020): 0233323.
- Ferré C, Callaghan W, Olson C, et al. Effects of maternal age and age-specific preterm birth rates on overall preterm birth rates—United States, 2007 and 2014. Morbidity and Mortality Weekly Report 65 (2016): 1181-1184.
- Sohn K. The trend in the relationship of advanced maternal age to preterm birth and low birthweight. The European Journal of Contraception & Reproductive Health Care 22 (2017): 363-368.
- Fuchs F, Monet B, Ducruet T, et al. Effect of maternal age on the risk of preterm birth: A large cohort study. PloS one 13 (2018): 0191002.
- Keiser AM, Salinas YD, DeWan AT, et al. Risks of preterm birth among non-Hispanic black and non-Hispanic white women: Effect modification by maternal age. Paediatric and perinatal epidemiology 33 (2019): 346-356.
- Liu B, Xu G, Sun Y, et al. Association between maternal pre-pregnancy obesity and preterm birth according to maternal age and race or ethnicity: a population-based study. The Lancet Diabetes & Endocrinology 7 (2019): 707-714.
- Souza RT, Costa ML, Mayrink J, et al. Clinical and epidemiological factors associated with spontaneous preterm birth: a multicentre cohort of low risk nulliparous women. Scientific reports 10 (2020): 1-10.
- Da Silva AA, Simões VM, Barbieri MA, et al. Young maternal age and preterm birth. Paediatric and perinatal epidemiology 17 (2003): 332-339.
- Hollowell J, Oakley L, Kurinczuk JJ, et al. The effectiveness of antenatal care programmes to reduce infant mortality and preterm birth in socially disadvantaged and vulnerable women in high-income countries: a systematic review. BMC pregnancy and childbirth 11 (2011): 13.
- Wagura P, Wasunna A, Laving A, et al. Prevalence and factors associated with preterm birth at kenyatta national hospital. BMC pregnancy and childbirth 18 (2018): 1-8.
- Mrisho M, Obrist B, Schellenberg JA, et al. The use of antenatal and postnatal care: perspectives and experiences of women and health care providers in rural southern Tanzania. BMC pregnancy and childbirth 9 (2009): 10.