Criteria for the Evaluation of Workflow Management Systems for Scientific Data Analysis
Article Information
Aleyna Dilan Kiran1, Mehmet Can Ay2,3, and Jens Allmer4*
1Bioengineering, Faculty of Engineering, Ege University, Izmir, Turkey
2Molecular Biology and Genetics, Faculty of Science, Izmir Institute of Technology, Izmir, Turkey
3Life Science Informatics, University of Bonn, Bonn, Germany
4Medical Informatics and Bioinformatics, MST, Hochschule Ruhr West, Mülheim adR, Germany
*Corresponding author: Jens Allmer. Medical Informatics and Bioinformatics, MST, Hochschule Ruhr West, Mülheim adR, Germany
Received: 03 May 2023; Accepted: 10 May 2023; Published: 02 June 2023
Citation: Aleyna Dilan Kiran, Mehmet Can Ay and Jens Allmer. Criteria for the Evaluation of Workflow Management Systems for Scientific Data Analysis Journal of Bioinformatics and Systems Biology. 6 (2023): 121-133.
Share at FacebookAbstract
Many scientific endeavors, such as molecular biology, have become dependent on big data and its analysis. For example, precision medicine depends on molecular measurements and data analysis per patient. Data analyses supporting medical decisions must be standardized and performed consistently across patients. While perhaps not life-threatening, data analyses in basic research have become increasingly complex. RNAseq data, for example, entails a multi-step analysis ranging from quality assessment of the measurements to statistical analyses. Workflow management systems (WFMS) enable the development of data analysis workflows (WF), their reproduction, and their application to datasets of the same type. However, far more than a hundred WFMS are available, and there is no way to convert data analysis WFs among WFMS. Therefore, the initial choice of a WFMS is important as it entails a lockin to the system. The reach in their particular field (number of citations) can be used as a proxy for selecting a WFMS, but of the about 25 WFMS we mention in this work, at least 5 have a large reach in scientific data analysis. Hence other criteria are needed to delineate among WFMS. By extracting such criteria from selected studies concerning WFMS and adding additional criteria, we arrived at five critical criteria: reproducibility, reusability, FAIRness, versioning support, and security. Another five criteria (providing a graphical user interface, WF flexibility, WF scalability, WF shareability, and computational transparency) we deemed important but not critical for the assessment of WFMS. We applied the criteria to the most cited WFMS in PubMed and found none that support all criteria. We hope that suggesting these criteria will spark a discussion on what features are important for WFMS in scientific data analysis and may lead to developing WFMS that fulfill such criteria.
Keywords
Scientific data analysis workflows, WF evaluation criteria, WF reproducibility, WF reusability, FAIR Data analysis, WF scalability, WF security, WFMS
Scientific data analysis workflows articles; WF evaluation criteria articles; WF reproducibility articles; WF reusability articles; FAIR Data analysis articles; WF scalability articles; WF security articles; WFMS articles
Scientific data analysis workflows articles Scientific data analysis workflows Research articles Scientific data analysis workflows review articles Scientific data analysis workflows PubMed articles Scientific data analysis workflows PubMed Central articles Scientific data analysis workflows 2023 articles Scientific data analysis workflows 2024 articles Scientific data analysis workflows Scopus articles Scientific data analysis workflows impact factor journals Scientific data analysis workflows Scopus journals Scientific data analysis workflows PubMed journals Scientific data analysis workflows medical journals Scientific data analysis workflows free journals Scientific data analysis workflows best journals Scientific data analysis workflows top journals Scientific data analysis workflows free medical journals Scientific data analysis workflows famous journals Scientific data analysis workflows Google Scholar indexed journals WF evaluation criteria articles WF evaluation criteria Research articles WF evaluation criteria review articles WF evaluation criteria PubMed articles WF evaluation criteria PubMed Central articles WF evaluation criteria 2023 articles WF evaluation criteria 2024 articles WF evaluation criteria Scopus articles WF evaluation criteria impact factor journals WF evaluation criteria Scopus journals WF evaluation criteria PubMed journals WF evaluation criteria medical journals WF evaluation criteria free journals WF evaluation criteria best journals WF evaluation criteria top journals WF evaluation criteria free medical journals WF evaluation criteria famous journals WF evaluation criteria Google Scholar indexed journals WF reproducibility articles WF reproducibility Research articles WF reproducibility review articles WF reproducibility PubMed articles WF reproducibility PubMed Central articles WF reproducibility 2023 articles WF reproducibility 2024 articles WF reproducibility Scopus articles WF reproducibility impact factor journals WF reproducibility Scopus journals WF reproducibility PubMed journals WF reproducibility medical journals WF reproducibility free journals WF reproducibility best journals WF reproducibility top journals WF reproducibility free medical journals WF reproducibility famous journals WF reproducibility Google Scholar indexed journals WF reusability articles WF reusability Research articles WF reusability review articles WF reusability PubMed articles WF reusability PubMed Central articles WF reusability 2023 articles WF reusability 2024 articles WF reusability Scopus articles WF reusability impact factor journals WF reusability Scopus journals WF reusability PubMed journals WF reusability medical journals WF reusability free journals WF reusability best journals WF reusability top journals WF reusability free medical journals WF reusability famous journals WF reusability Google Scholar indexed journals FAIR Data analysis articles FAIR Data analysis Research articles FAIR Data analysis review articles FAIR Data analysis PubMed articles FAIR Data analysis PubMed Central articles FAIR Data analysis 2023 articles FAIR Data analysis 2024 articles FAIR Data analysis Scopus articles FAIR Data analysis impact factor journals FAIR Data analysis Scopus journals FAIR Data analysis PubMed journals FAIR Data analysis medical journals FAIR Data analysis free journals FAIR Data analysis best journals FAIR Data analysis top journals FAIR Data analysis free medical journals FAIR Data analysis famous journals FAIR Data analysis Google Scholar indexed journals WF scalability articles WF scalability Research articles WF scalability review articles WF scalability PubMed articles WF scalability PubMed Central articles WF scalability 2023 articles WF scalability 2024 articles WF scalability Scopus articles WF scalability impact factor journals WF scalability Scopus journals WF scalability PubMed journals WF scalability medical journals WF scalability free journals WF scalability best journals WF scalability top journals WF scalability free medical journals WF scalability famous journals WF scalability Google Scholar indexed journals WF security articles WF security Research articles WF security review articles WF security PubMed articles WF security PubMed Central articles WF security 2023 articles WF security 2024 articles WF security Scopus articles WF security impact factor journals WF security Scopus journals WF security PubMed journals WF security medical journals WF security free journals WF security best journals WF security top journals WF security free medical journals WF security famous journals WF security Google Scholar indexed journals WFMS articles WFMS Research articles WFMS review articles WFMS PubMed articles WFMS PubMed Central articles WFMS 2023 articles WFMS 2024 articles WFMS Scopus articles WFMS impact factor journals WFMS Scopus journals WFMS PubMed journals WFMS medical journals WFMS free journals WFMS best journals WFMS top journals WFMS free medical journals WFMS famous journals WFMS Google Scholar indexed journals RNA-seq articles RNA-seq Research articles RNA-seq review articles RNA-seq PubMed articles RNA-seq PubMed Central articles RNA-seq 2023 articles RNA-seq 2024 articles RNA-seq Scopus articles RNA-seq impact factor journals RNA-seq Scopus journals RNA-seq PubMed journals RNA-seq medical journals RNA-seq free journals RNA-seq best journals RNA-seq top journals RNA-seq free medical journals RNA-seq famous journals RNA-seq Google Scholar indexed journals
Article Details
1. Introduction
Many disciplines, such as physics and medicine, include data-driven endeavors. In the latter field, precision medicine is gaining interest. Precision, or personalized, medicine depends on measurements at the molecular level for patient stratification. Similarly, in many fields of biology, molecular measurements have become indispensable. Such measurements, however, present a formidable data analysis challenge. Even seemingly simple questions, such as which RNA is expressed in a sample and which one is not, entail multi-step data analysis workflows (WF) ranging from quality assessment of the raw data to statistical significance analysis. Despite such guidance as provided by Conesa et al. [1], many current works utilize idiosyncratic approaches to RNA-seq data analysis. The data analysis workflows used are sometimes provided as a supplement to a publication but often are only described on a relatively high-level, hampering reproducibility. For the underlying data, it has been recognized that they should be available not only for the reproduction of the results but also for integrative analyses and other data analysis purposes. The FAIR [2] principles, findable, accessible, interoperable, and reusable, describe essential aspects that need to be considered in data governance. One crucial part is finding the data, which can be achieved via a digital object identifier (DOI) and appropriate annotation or metadata. With growing adherence to the FAIR principles, reproducibility is no longer hampered by data availability. It is time to apply similar principles to the data analysis workflows used to (re-)interpret FAIR data. Scientific data analysis WFs come in many flavors, ranging from custom-written code (small applications) for data analysis to WFs created in workflow management systems (WFMS) resembling visual programming. Custom software to solve a particular data analysis challenge may be the least FAIR, in analogy to the FAIR principles, as it is not guaranteed to be findable. However, some journals may require a link to a public repository such as GitHub [3]. Still, GitHub repositories may not be maintained after a while, and they might be closed by the owner so that the custom software is no longer accessible. Interoperability involves a considerable effort, too much for typically short-lived exploratory data analysis endeavors. In our experience, the reproducibility of such code is very low, and a great effort is needed to execute in a different environment, only to find that the results are largely different from other similar tools.
Better approaches are tools that can also define how dependent software is to be installed, such as Chef [4], SnakeMake [5], or other make tools. However, make tools are often not used to their potential and are riddled with calls to local files, which makes them hard to use in a different environment and strongly hamper reproducibility. Dedicated WFMS with a graphical user interface resemble visual programming approaches and typically restrict the modules that can be used to a set they make available with their tool, in a tool shed, or in a repository. This approach increases reproducibility and interoperability, at least within the same WFMS. These tools typically enable sharing of workflows and their findability so that the FAIR principles for data are at least approximated at this level. Of course, attempting interoperability among WFMS must remain a futile undertaking. General interoperability has been considered [6], but attempts seem to have failed. However, a handful of approaches try to make two WFMS interoperable, such as the common workflow language (CWL) [7]. Still, among the tools considered here, only Pegasus [8] and Galaxy [9] have partial CWL implementations, and the reference implementation for CWL, cwltool [10], is not widely used, judging by its few references in PubMed.
While we are aware that no method is best suited for all instances of a problem, we wondered why there were so many WFMS (Table 1). The WFMS in Table 1 are not equally used in science, judging by the number of citations. However, approximately ten WFMS seem to have a considerable user base. We have created custom WFs and targeted data analysis applications in the past and later switched to WFMS, such as KNIME [11], Galaxy [9], Cuneiform [12], and CLC [13]. Comparing the many WFMS is extremely time-consuming, and considering the low number of citations for some WFMS does not seem indicated. Hence there is no guidance for users that wish to use a WFMS to improve their data analysis WFs. To address this issue, we scanned the literature for manuscripts discussing WFMS in general or comparing some WFMS. We selected eight studies and integrated the ideas they discussed to arrive at the first list of features critical for WFMS in scientific data analysis. We compiled this list and defined our understanding of these features as the first reference. We then applied it to the most used WFMS in scientific practice. None of the WFMS fully supported the critical features on their most advanced levels according to the definitions we provide. With this manuscript, we aim to open a discussion of feature definitions, critical, important, and nice-to-have features and hopefully see the development of WFMS that provides these features. This is critical in order to trust data analysis results and apply them in critical areas such as precision medicine.
1.1 Workflow Management Systems
For our discussion on data analysis platforms in science, we start by acknowledging that it is impossible even to screen a small part of the data analysis pipelines that have been used in a very confined area, such as bioinformatics analysis of mass spectrometry (MS)-based proteomics data. In the past, pipelines in MS-based proteomics were often hard-coded scripts, including calls to locally installed software. Improvements came with platforms such as Open-MS [14], which first provided a tool base and later a dedicated WFMS (TOPP [15]) for MS-based analysis tasks. This example can be found similarly in many areas of scientific data analysis. The last decade saw a strong increase in the development and use of broader workflow management systems such as Galaxy and KNIME. In our analysis, we ignore all targeted systems and only consider WFMS that are broadly applicable. Searching the web, Google Scholar, and PubMed, we compiled a list of WFMS applied for data analysis in science (Table 1). However, far more than a hundred WFMS exist, but only very few have a relevant number of references warranting their mention in this setting.
Table 1: Workflow management systems used for data analytics in science. The number of citations is determined by searching PubMed with suitable search terms in early 2022. More comprehensive lists of WFMS can be found in on (GitHub: https://github.com/common-workflow-language/common-workflow-language/wiki/Existing-Workflow-systems and https://github.com/pditommaso/awesome-pipeline.) Status describes whether the WFMS is open or closed source, commercial or freely available (public).
![]() |
With the availability of hundreds of WFMS and still tens of WFMS referenced in PubMed (Table 1), the choice of WFMS is not obvious since criteria such as the number of citations can be very misleading, confounded, for example, by the publication date and the professional networks of the developers. Therefore, we set forth to collect features needed in scientific data analysis of WFMS, defined them as precisely as we could, and suggested criteria for evaluating WFMS.
1.2 Establishment of Criteria for Workflow Management System Evaluation
Many workflow management systems have been developed and are used in scientific data analysis (Table 1). Some of the WFMS are cited more frequently than others which may indicate that they are used more often or merely shows that they are more available or better advertised. Currently, there are no other criteria to evaluate the fit of a WFMS to the scientific data analysis task than the citations in the field. Therefore, we decided to take the initiative and suggest several criteria for evaluating WFMS for scientific data analysis.
As a first step, we screened the literature for reviews concerning WFMS, but only a handful of works discussed features of WFMS. The selected papers are Beukers and Allmer [31], Wratten et al. [32], Sharma et al. [33], Karim et al. [34], Verhoeven et al. [35], Jackson et al.(Jackson et al., 2021), Larsonneur et al. [36], and Lamprecht et al. [37].
For example, Wratten et al. focus their discussion of WFMS features on the ease of use, expressiveness, portability, scalability, availability of learning resources, and pipeline initiatives for the WFs.
We then extracted features from the selected papers and compiled them into a list which we later consolidated by grouping and generalizing our naming of the features. The complete list of features is available in Supplementary
Table S1. We defined the selected features such that their support in the current WFMS can be evaluated, considering multiple levels for the various features. With the consolidated list of features and our definitions, we counted their support within the selected manuscripts (Table 2). Simply picking the features for WFMS in decreasing support order would be possible. However, some features seem critical, but they do not have high support, which may be due to many reasons, including that authors implicitly take the features for granted or do not consider applications concerning, for example, personal data. Security is such a feature that, although it has almost no support, we deem it extremely critical, especially when handling patient data. Versioning is another feature with low support critical to ensure reproducibility and ease the implementation of cooperative tasks.
The high support for a graphical user interface (GUI) is on the other side of the spectrum. Clearly, having a GUI is of no consequence for reproducibility or some other of the truly critical features.
Table 2: Feature consensus Consensus scores are calculated by dividing the number of articles mentioning the criteria by the total number of articles. Here we only present the ten selected critical and important features, but a more comprehensive consensus is available in Supplementary Table S1.
![]() |
By decreasing consensus, we offer the following features for discussion as viable criteria for the evaluation of workflow management systems, 1) reproducibility, 2) reusability, 3) FAIRness, 4) versioning support, and 5) security. The next five criteria we find important but not critical are 1) providing a graphical user interface, 2) allowing for WF flexibility, 3) concerning WF scalability, 4) WF shareability, and 5) computational transparency. With this list of critical and important features and how they were used in the manuscripts we considered, we developed definitions for the terms trying to include multiple levels for each criterion.
1.3 Definition of Criteria for Scientific Data Analysis Workflows
We selected several review articles and articles discussing various aspects of workflow management systems. From these works, we compiled a list of features that a WFMS should have or that are associated with scientific data analysis WFs. In the following, we explain the critical and important features. Clearly, these definitions are subjective, and we hope to spark a debate by providing these first definitions. The definitions are provided in the same order as above, with the critical features first followed by important features in decreasing order of consensus where possible.
1.4 Critical Criteria
Reproducibility is the ability to generate the same result with the same dataset using the same workflow with identical parameter settings, regardless of other environment choices, such as the operating system (OS). This definition is more stringent than its typical meaning in science, but the expectation is that correct algorithms will be employed for the data analysis of the same data with the same settings. In this case, the results must be identical and not just close as could be expected in a biological experiment.

Figure 1: Basic reproducibility of data analysis workflows.
Aiming to transfer the workflow to others or among WFMS is covered under reusability and shareability. Reproducibility increases with well-tested WFMS and equally well-tested WFs, which is covered under versioning and reliability. Exact reproducibility must be ensured for all components of a WF, not only the overall WFMS since some components could be external or could call external processes. With these restrictions in mind, one question that needs to be asked is how the data processing is performed within the workflow. If 1) the calculations are performed via tools that are part of the WFMS, then reproducibility could be expected. If 2) the analyses are performed by automatically discoverable and installable addons via the WFMS, reproducibility could be expected. If 3) the calculations are performed by third-party tools, then the WFMS needs a mechanism to discover whether tools are installed locally and install them if needed.
- Processing nodes are part of the WFMS core,
- Addons can be automatically discovered and installed,
- The local availability of third-party tools can be discovered, and tools can be installed automatically if needed.
Reusability defines the ability to reuse the WF for the intended purpose or a related one. The most basic reusability ensures that the WF can be used with different data of the same type in the same WFMS on the same machine. The second step is enabling the sharing of the workflow with other users of the same WFMS (also see shareability). This could be supported by platforms that provide access to the WFs via DOIs, like FAIR data. The third step is to support platform-independent sharing, as mentioned by Wratten and colleagues [32]. This third step seems elusive due to the large number of platforms and the previous attempts at platform independence, such as Shiwa [6]. These three aspects only cover the reuse of the WF with different data but the same analysis intention. Another critical aspect of reusability is how narrow the focus of the WF is and whether it can be employed for related types of analysis. The FAIR principles also aim to enable re-analysis of data with different perspectives than the one of the data producers. Here the question is whether WFs can be used for only one analysis, such as the alignment of single-end RNA-seq data from an Illumina measurement, or whether it could accept different types of RNA-seq data measured with machines from other vendors. Another interesting question under reusability is whether parts of the WF can be reused. For example, performing a statistical analysis should be relatively agnostic of the domain and could be reused in many WFs. KNIME, for instance, can create different meta-nodes encapsulating sub-workflows. Such modules lend themselves to easy reuse within different WFs. In summary, reusability covers three aspects:
- Use of the WF to analyze different data of the same type with the same intention,
- Use of the WF to analyze somewhat different data with different intentions,
- Use of parts of the WF to build new WFs.
FAIR is an initiative to make scientific data findable, accessible, interoperable, and reusable [2]. Journals often enforce the findable and accessible parts of FAIR during the publication of manuscripts that include generated data by requiring its deposition in publicly accessible repositories. For data, interoperability can be achieved by using a standard representation of such data, such as mzML in proteomics [38]. The reuse of data typically depends on the level of documentation and the metadata that was collected. The better data collection and data are annotated, the higher its reusability for other purposes.
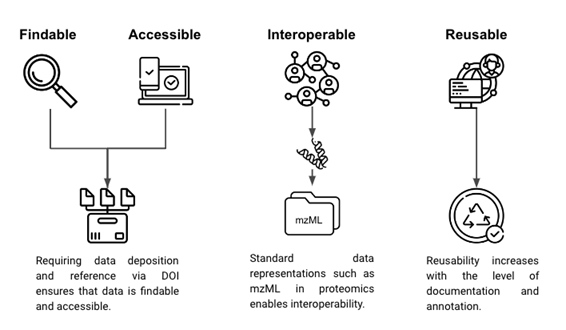
Figure 2: Findable, accessible, interoperable, and reusable.
For WFs, reusability is covered above, and findable and accessible tie in with shareability below. We covered interoperability as part of reusability above, as well. Perhaps the interoperability issue for Workflows could be reformulated such that it would become a data standardization and general automation issue. In this way, WFs can be viewed as pipes that accept a certain type of input and generate a particular output. Both in- and output should be community-accepted standards. Then these WFs can be run in their specific WFMS, and the OS can be employed to start the WFMS and WF as soon as data becomes available. The downside is that many WFMS with their dependencies may have to be installed and that all components need to be trustworthy (i.e., on a very high technology readiness level).
Jackson and colleagues put forth the notion that WFs should be open source [39]. We fully agree with this idea and would like to extend it such that the FAIR principles should also be applied to data analysis workflows. FAIR data also ensures that data that underpins a scientific finding can be checked for tampering and that any subsequent data analysis builds on the same data. Making WFs FAIR has similar effects. The data analysis can be directly checked for any methodological problems, and the same WF can be used for reproduction and reuse.
Versioning (collaboration, coauthoring) is the ability to track modifications made to a WF within a WFMS. Both change and editor should be trackable similar to versioning systems such as Git. Stable versions need to be identifiable so that FAIRness is ensured. With current systems, versioning extends further to the underlying tools, whose versions may not always be tracked or may not be trackable by the WFMS. Often, WFMS provide access to command line tools produced by third parties. Swapping out one version of such third-party tools against another may significantly change the data analysis results. Hence these versions also need to be tracked. WFMS also undergo changes, and it must be clear how to access stable versions to combine the right tools with the fitting WF and WFMS versions. In short, we identified three areas that would benefit from versioning:
- Versions of the underlying tools used in a WF,
- Versions of a WF,
- Versions of the WFMS.
Security is the ability to protect access to the dataset, WF, and the outcome of the workflow that should only be accessible to verified individuals. For example, healthcare providers should protect patients' medical data since they may contain personal information and can be used against identifiable individuals. Companies likewise need security as they may want to develop a commercial product, so access needs to be restricted before release or for paying customers. Military applications, even those developing vaccines or treatment options in case of attacks with bioweapons, need special security. In medicine, the workflow should often be open access but the input and output data only under specific conditions. Of course, this can be achieved by operating in a secure environment, but that hinders collaboration. If a WFMS were to be used, not only must the WFMS be secure, but all components, e.g., third-party tools that are automated within the WFMS, must have strong security in place. Third-party tools may include deliberate backdoors or may not be designed to withstand hacking. We define four levels of security:
- The WFMS is secure,
- User authentication is in place,
- Security of the components making up workflows is guaranteed,
- The possibility to restrict access to WFs and data with fine granularity.
Sharma et al. mentioned security as an important issue that needs to be addressed [33]. However, they provide no further information, and other works do not mention security.
1.5 Important Criteria
Graphical user interfaces (GUI) can be understood as any visual interaction with a computer system. Hence making files would qualify as applications with a GUI. We would instead like to refer to the visual development of pipelines via point-and-click when defining a GUI. However, more than this may be needed, and adding presentation facilities for other stakeholders without IT skills is necessary for a complete GUI. Finally, the workflow execution should be visible (see transparency) in the GUI. That means a GUI should cover all aspects within a WFMS,
- WF development,
- WF presentation, and
- Transparent WF execution.
Flexibility/Expressiveness refers to how ideas can be implemented into workflows. This criterion represents a tradeoff between what can be expressed and how well human readers can fully understand it. For example, the availability of branching, looping, and encapsulation for workflows affords higher expressiveness but simultaneously reduces the ability of users to comprehend the workflows quickly and easily. With an increase of different technologies for the same purpose (e.g., next-generation sequencing platforms), workflows need to be generated for each platform if the WFMS has no branching capabilities. Otherwise, tests to automatically determine the platform from input data can be incorporated into the workflow. These tests could be further encapsulated in sub-workflows so that the overall workflow remains easily understandable to users. While allowing for only a unidirectional flow of information without branching and looping makes WFs more comprehensible, higher expressiveness is called for by the current needs for WF development. At this point, we are looking for four constructs to assess the expressiveness of a WFMS with the 5th feature, free data flow, probably creating very high theoretical expressiveness but very low comprehensibility of the workflow.
- Variables,
- Encapsulation (also adding scoping for variables),
- Branching,
- Looping (perhaps also allowing recursion),
- Free data flow.
Scalability refers to the ability of a WFMS to allow a WF to process large amounts of data seamlessly. There are at least two types of scaling, horizontal and vertical. Horizontal scaling can, for example, be achieved by executing the workflow in parallel on many processing nodes (e.g., cloud computing). Vertical scaling is also important in scientific computing since some processes depend on particular resources, e.g., memory, that are typically limited in high-performance computing (HPC) environments. Vertical scaling is usually trivial by just executing the WF on a computer resource with the required specifications. On the other hand, horizontal scaling may involve communication with the HPC submission system and collecting the results. Submission to an HPC can also be performed differentially for each calculation step in a WF.
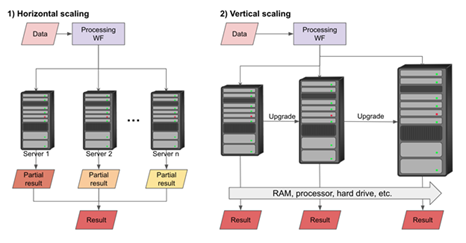
Figure 3: Two ways of scaling data analysis workflows.
The WF provider could associate machine requirements with each calculation step, or a central database could have this information. However, HPC clusters and their submission systems are diverse, and there is no standard to represent these dependencies. The latter task is different for each HPC system and thereby poses challenges to WFMS. In addition, the efficiency, cost of computing, and handling of failed execution attempts should be considered, which makes scaling a formidable challenge for WFMS.
Multiple levels of scalability can be considered:
Workflows can be virtualized or turned into an executable which users can then run in their HPC (e.g., WF packaged in Docker (https://www.docker.com) containers) [40],
- WFMS automatically package the WF and execute it on a specific HPC environment, such as Slurm [41] or HTC Condor [42],
- WFMS directly submits each processing step differentially to a specific HPC environment,
- WFMS directly submits each processing step differentially to any configurable HPC environment,
- WFMS considers efficiency and cost with the submission process and handles failed execution attempts.
Shareability
Sharing a workflow can mean that a code artifact is provided, which is executed elsewhere. We believe that this is not enough and define multiple levels of shareability. On the first level, it must be ensured that the workflow can be executed in the same WFMS installed in a different system. This is typically possible with WFMS but not necessarily with make files unless they are very carefully implemented. The next level of sharing involves platforms that enable the distribution of a WF among users of the WFMS, implementing findability. There are examples such as nf-core [43] (<100 WFs) for NetFlow, KnimeHub [44] (>11000 WFs) for KNIME, and many more. A platform allowing WFMS agnostic sharing of such platforms is the gold standard at this level which has existed for a long time as myExperiment [45] (~3000 WFs). On the next level, shareability ties in with reusability by requiring that the WFs can also be converted or directly executed among platforms. Apart from these three levels is the curation of WFs. This curation should have two pillars. One is manual curation by the community, not by self-appointed experts, so a feedback system needs to be in place. The other is continuous testing of the WFs on synthetic and experimental data with known outcomes. Whenever errors occur, all stakeholders should be informed. Stakeholders are, for example, users of the WF but also other developers of WFs, which may depend on this WF. In summary shareability of WFs means to us that WFs
- can be distributed and executed,
- are shared in an accessible repository and can be executed,
- can be shared among environments and executed in any of them,
- are continuously curated, tested, and problems are communicated to all dependents and users of a tool or WF.
Wratten et al. [32] define portability as the integration of the WFMS with containers and package managers. We include this more abstractly in shareability and refrain from commenting on using containers and package managers. However, containers and package managers entail a completely different set of problems, such as allowing WFs to use an outdated version of a tool, thereby ignoring potentially crucial bug fixes with no current communication system in place.
Computational transparency refers to the transparency of the execution of the data analysis steps. For example, it should be apparent where the computations are performed and how long they will take on the given hardware. The former also plays a role in security, and the latter is important for workflow sharing, as the computational power of the hardware may be crucial for some calculations (see scalability).
Storage or caching of intermediate results and frequently used files are other important features of computational transparency. Together they can aid re-entrancy [33], which saves significant amounts of computing time should a WF be disrupted during execution. KNIME, for example, has a traffic light system where red indicates a processing step that still needs configuring. Yellow shows that the processing step can be executed, and green signifies that it has been successfully completed.
Karim et al. used the term computational transparency to refer to the transparency of the data analysis [34]. We cover the different aspects of transparency of data analysis within versioning, FAIR, reliability, and reusability.
1.6 Criteria Application
We defined five critical and five important criteria for the evaluation of WFMS. An immediate question is how far the current WFMS fulfill these criteria. For many of the criteria, we define several levels so that we can determine not only whether a criterion is fulfilled but also at which level. Answering this question for all available WFMS is beyond the scope of this work, and therefore, we selected the five most cited WFMS in PubMed. The criteria are ordered by consensus score and the WFMS by the total number of citations (Table 3). One critical criterion is reproducibility. All WFMS considered can execute the same WF with the same data in the same environment. However, if calculations are deferred to tools not part of the WFMS core, it could be a problem if even the OS is reinstalled since dependencies may not be present under those circumstances. KNIME can automatically discover missing modules and offers their automatic installation. No WFMS in this list can automatically test whether all dependencies on third-party tools are fulfilled, install such tools automatically, or employ tools such as Chef to invoke installation scripts.
Reusability is a criterion that is not only dependent on the WFMS but also on the WF. If the WF is constructed such that input files are hardcoded, which is possible in all of the WFMS, then reusability is reduced. All WFMS afford reusability at the primary level, and KNIME also supports encapsulation and reuse of parts of a WF. Galaxy also offers encapsulation, but we have faced problems with this in practice [31].
An important part of FAIRness is enabling the finding of a WF. This is possible independent of WFMS when depositing the WF in myExperiment. Such a central repository would be the best approach, but WFMS often include a sharing platform for WF developed in their platform. The latter presents a hindrance for FAIRness since many platforms must be searched, which may have different access criteria, and most likely will not allow interoperability. myExperiment or something similar should become part of EBI, NCBI, and similar institutions to provide a central repository for WFs as exists for data such as the sequence read archive [46].
Table 3: State of integration of chosen criteria into different workflow management systems. We indicate the level of the criteria that we believe are being reached by the WFMS. Please note that this assignment is optimistic and that we assign the highest possible level of the criteria in case it seems covered.
Tool Name / Criteria |
Reproducibility |
Reusability |
FAIRness |
Versioning |
Security |
KNIME |
2 |
1, 2, 3 |
KNIME hub |
1#, 2, 3 |
1, 2, 3x, 4 |
as a commercial add-on |
for the commercial server |
||||
GenePattern |
0 |
1 |
0, code is generated; e.g., Java |
1#, 2, 3 |
1, 2, 4 |
Galaxy |
0 |
1 |
Galaxy Toolshed |
2, 3 |
1, 2 |
Pegasus |
0 |
1 |
0, few selected on the website |
3 |
1, 2 |
Unipro UGENE |
0 |
1 |
? |
3 |
1, 2, 4* |
SnakeMake |
0 |
depends on make-file |
depends on the developer |
depends on the developer |
depends on the developer |
Nextflow |
0 |
1 |
integration with GitHub |
3 |
1, 2, 4 |
CLC bio |
1 |
1 |
0 |
1, 2, 3 |
1, 2, 3 |
x In case only core nodes are used, security is probably high, but this cannot be said for third-party tools, especially non-trusted ones.
* When using UGENE, shared database security is unclear to us.
# Only applies to tools included in the platform and not to automated third-party tools.
All popular WFMS lack in the most important criterion, which is reproducibility. Here it is important that even given the same WFMS, executing a given workflow on a different computer, let alone in a different operating system, is not trivial. Part 2 of the criterion reproducibility, automatic detection, and installation of add-ons was only available in KNIME. However, this cannot be taken for granted for all nodes, especially third-party nodes dealing with bioinformatics. For example, when using R-scripts with KNIME, users are fully responsible for installing R and all dependent libraries. The same is true for third-party tools such as FastQC that could be automated with KNIME WFs.
A similar scenario to reproducibility emerges for reusability. Most WFMS can be used to analyze different datasets with the same workflow and the same intention, but as soon as the input differs, WFs would need to be more complex and include decision-making. This would be possible with KNIME, where branching and looping is supported. Reuse of sub-workflows can also be achieved in KNIME, especially when using the commercial KNIME server.
The criteria versioning and security are in a better position because developing such WFMS includes versioning systems and access restrictions so that these mechanisms are naturally integrated into the WFMS. One important issue is that many WFMS only automate tools such as Bowtie [47]. Thereby, versioning and security do not extend to the actual building blocks of WFs.
SnakeMake is a popular tool for implementing data analysis WFs, but most criteria we consider depend on the specific WF rather than the platform itself. Hence, for each WF produced with SnakeMake, the criteria must be applied, which is cumbersome.
Applying the critical criteria to the most popular WFMS was instructive, but there are many more questions that could and should be asked before settling for a WFMS. A critical question is which processors (nodes) are available and whether it is possible to get timely help.
Further Important Questions
Documentation and Help
Other features that differentiate among WFMS are documentation, example workflows, help desks, and other means of ensuring user productivity. KNIME has the KNIME Hub with example workflows, comprehensive documentation, and help on various levels. Most useful to us, however, is the third-party tool NodePit [48] which analyzes node usage statistics and provides examples of how nodes are typically assembled into WFs. This structured approach is not available for any other WFMS but would be a nice feature for myExperiment.
Available Processors
There is an inflation of tools in bioinformatics, and many tools for the same purpose exist. For example, there are at least 50 tools that perform read mapping. While only a few tools have a significant reach in the community, even these have not been compared comprehensively. This is one example of many, and while this is not the topic of this work, it is important to keep in mind that interchanging tools that seemingly perform the same action within a data analysis WF will typically change the results (sometimes drastically). Another important point is that the technical correctness of the tools is typically not proven and that independent testing of these tools is rare.
2. Conclusions
Biology and medical sciences are becoming more and more data-driven endeavors. With the advent of precision medicine, this data dependence will grow further. This increase in data entails a need for a significant effort in data analysis. Today data analysis is often performed using workflow management tools. These tools allow data analysis workflows to be established once and then reapplied to similar measurements. Many workflow management tools have been established, and we list a small subset of around 20 such systems in Table 1. We have previously shown that it is hard to recreate the same data analysis workflow in several workflow management tools [31]. Sharing of workflows among platforms is currently only supported for very specific scenarios. There were efforts such as Shiwa [6] aiming to make workflows sharable among platforms, but such endeavors must remain futile considering the vast amount of WFMS.
Therefore, choosing a workflow management platform is important. However, platforms have largely different workflow management approaches and very different toolsets. At the same time, comparisons of such platforms are rare, and evaluation criteria are not established. We set forth to remedy the latter by providing a set of criteria for the comparison of workflow management systems which is, at the same time, a wish list for features of future platforms. The list has been compiled by reviewing the available, albeit scarce, literature and choosing frequently mentioned features. While we formed a consensus among the studies (Table 2), we did not strictly pick the features according to the consensus score.
Ordered by decreasing consensus score, we offer the following criteria for discussion as critical criteria for evaluating workflow management systems, 1) reproducibility, 2) reusability, 3) FAIRness, 4) versioning support, and 5) security. The following five criteria we find important but not critical: 1) providing a graphical user interface, 2) allowing for WF flexibility, 3) concerning WF scalability, 4) WF shareability, and 5) computational transparency. The greatest consensus is that a WFMS should have a graphical user interface for WF construction. However, in our opinion, it is not a critical feature for WFMS.
Applying the critical criteria to the most cited WFMS in PubMed shows that current WFMS only support these features partially, and none fulfills all criteria (Table 3). However, these critical features may impact scientific findings, interpretation, and communication of results. By offering these criteria, we hope to jumpstart the discussion on comparing WFMS and initiate the creation of a consensus list of features that future WFMS should include.
Ethical Approval and consent to participate
Not applicable.
Consent for Publication
Not applicable.
Data Availability statement
All data is contained within the publication or its supplement.
Conflict of interest
The authors declare no conflict of interest.
Funding
The authors received no funding for their work.
Acknowledgment
Not applicable.
Author contribution
JA developed the study and performed a part of the research. ADK and MCA performed research and prepared tables and figures. All authors prepared the manuscript collaboratively. JA performed the final editing. All authors read and approve the final manuscript.
References
- Conesa A, Madrigal P, Tarazona S, et al. A survey of best practices for RNA-seq data analysis. Genome Biol 17(2016): 13.
- Wilkinson MD, Dumontier M, Aalbersberg IjJ, et al. The FAIR Guiding Principles for scientific data management and stewardship. Sci Data 3(2016): 160018.
- (2022). https://github.com/.
- Chef Software DevOps Automation Solutions | Chef. https://www.chef.io/. Accessed 5 Jul 2022.
- Koster J, Rahmann S. Snakemake–a scalable bioinformatics workflow engine. Bioinformatics 28(2012): 2520–2.
- Korkhov V, Krefting D, Montagnat J, et al. SHIWA workflow interoperability solutions for neuroimaging data analysis. Stud Health Technol Inform 175(2012): 109–10.
- Amstutz P, Crusoe MR, Tijanic N, et al. Common Workflow Language, v1.0. United States: Figshare 10(2016).
- Deelman E, Vahi K, Juve G, et al. Pegasus, a workflow management system for science automation. Future Gener Comput Syst 46(2015): 17–35.
- Afgan E, Baker D, Batut B, et al. The Galaxy platform for accessible, reproducible and collaborative biomedical analyses: 2018 update. Nucleic Acids Res 46(2018): 537–44.
- Crusoe MR, Abeln S, Iosup A, et al. Methods Included: Standardizing Computational Reuse and Portability with the Common Workflow Language. Commun ACM 10(2022): 65.
- Berthold MR, Cebron N, Dill F, et al. KNIME: The Konstanz Information Miner. In: Preisach C, Burkhardt H, Schmidt-Thime L, Decker R, editors. Data Analysis, Machine Learning and Applications. Berlin, Heidelberg: Springer 10(2008): 319–26.
- BRANDT J, REISIG W, LESER U. Computation semantics of the functional scientific workflow language Cuneiform. J Funct Program 27(2017): 22–22.
- CLC Genomics Workbench. (2013).
- Sturm M, Bertsch A, Gröpl C, et al. OpenMS – An open-source software framework for mass spectrometry. BMC Bioinformatics 9(2008): 163–163.
- Kohlbacher O, Reinert K, Gröpl C, et al. TOPP--the OpenMS proteomics pipeline. Bioinforma Oxf Engl 23(2007): 191-197.
- Reich M, Liefeld T, Gould J, et al. GenePattern 2.0. Nat Genet 38(2006): 500–1.
- Okonechnikov K, Golosova O, Fursov M, et al. Unipro UGENE: a unified bioinformatics toolkit. Bioinforma Oxf Eng 28(2012): 1166–7.
- Di Tommaso P, Chatzou M, Floden EW, et al. Nextflow enables reproducible computational workflows. Nat Biotechnol 35(2017): 316–9.
- Hull D, Wolstencroft K, Stevens R, et al. Taverna: a tool for building and running workflows of services. Nucleic Acids Res 34(2006): 729-32.
- Cervera A, Rantanen V, Ovaska K, et al. Anduril 2: upgraded large-scale data integration framework. Bioinforma Oxf Engl 35(2019): 3815–7.
- Mierswa I, Wurst M, Klinkenberg R, et al. YALE. In: Proceedings of the 12th ACM SIGKDD international conference on Knowledge discovery and data mining - KDD ’06. New York, New York, USA: ACM Press 10(2006): 935–935.
- Gardeux V, David FPA, Shajkofci A, et al. ASAP: a web-based platform for the analysis and interactive visualization of single-cell RNA-seq data. Bioinforma Oxf Engl 33(2017): 3123–5.
- Elhai J, Taton A, Massar JP, et al. BioBIKE: a Web-based, programmable, integrated biological knowledge base. Nucleic Acids Res 37(2009): 28-32.
- Reisinger E, Genthner L, Kerssemakers J, et al. OTP: An automatized system for managing and processing NGS data. J Biotechnol 261(2017): 53–62.
- Wang Y, Mehta G, Mayani R, et al. RseqFlow: workflows for RNA-Seq data analysis. Bioinformatics 27(2011): 2598–600.
- Linke B, Giegerich R, Goesmann A. Conveyor: a workflow engine for bioinformatic analyses. Bioinformatics 27(2011): 903–11.
- Rowe A, Kalaitzopoulos D, Osmond M, et al. The discovery net system for high throughput bioinformatics. Bioinforma Oxf Engl 19(2003): 225-231.
- Lampa S, Dahlö M, Alvarsson J, et al. SciPipe: A workflow library for agile development of complex and dynamic bioinformatics pipelines. GigaScience 8(2019): 044.
- Ko G, Kim P-G, Yoon J, et al. Closha: bioinformatics workflow system for the analysis of massive sequencing data. BMC Bioinformatics 19(2018): 43–43.
- Scheidegger CE, Vo HT, Koop D, et al. Querying and re-using workflows with VsTrails. In: Proceedings of the 2008 ACM SIGMOD international conference on Management of data. New York, NY, USA: Association for Computing Machinery 10(2008): 1251–4.
- Beukers M, Allmer J. Challenges for the Development of Automated RNA-seq Analyses Pipelines. GMS Med Inform Biom Epidemiol 17 (2023).
- Wratten L, Wilm A, Göke J. Reproducible, scalable, and shareable analysis pipelines with bioinformatics workflow managers. Nat Methods 18(2021): 1161–8.
- Sharma A, Rai A, Lal S. Workflow management systems for gene sequence analysis and evolutionary studies - A Review. Bioinformation 9(2013): 663–72.
- Karim MR, Michel A, Zappa A, et al. Improving data workflow systems with cloud services and use of open data for bioinformatics research. Brief Bioinform 19(2018): 1035–50.
- Verhoeven A, Giera M, Mayboroda OA. Scientific workflow managers in metabolomics: an overview. Analyst 145(2020): 3801–8.
- Larsonneur E, Mercier J, Wiart N, et al. Evaluating Workflow Management Systems: A Bioinformatics Use Case. In: 2018 IEEE International Conference on Bioinformatics and Biomedicine (BIBM). Madrid, Spain: IEEE 10(2018): 2773–5.
- Lamprecht A-L, Palmblad M, Ison J, et al. Perspectives on automated composition of workflows in the life sciences. F1000Research 10(2021): 897.
- Deutsch EW. Mass Spectrometer Output File Format mzML. Methods Mol Biol Clifton NJ 604(2010): 319–31.
- Jackson M, Kavoussanakis K, Wallace EWJ. Using prototyping to choose a bioinformatics workflow management system. PLOS Comput Biol 17(2021): e1008622.
- Cheng G, Lu Q, Ma L, et al. BGDMdocker: a Docker workflow for data mining and visualization of bacterial pan-genomes and biosynthetic gene clusters. PeerJ 5(2017): e3948.
- Yoo AB, Jette MA, Grondona M. SLURM: Simple Linux Utility for Resource Management. In: Feitelson D, Rudolph L, Schwiegelshohn U, editors. Job Scheduling Strategies for Parallel Processing. Berlin, Heidelberg: Springer Berlin Heidelberg 12(2003): 44–60.
- Thain D, Tannenbaum T, Livny M. Distributed Computing in Practice: The Condor Experience. Concurr Comput Pract Exp 17(2005): 2--4.
- Ewels PA, Peltzer A, Fillinger S, et al. The nf-core framework for community-curated bioinformatics pipelines. Nat Biotechnol 38(2020): 276–8.
- KNIME, Team. KNIME Hub, Zurich, Zwitserland (2023). https://hub.knime.com/
- Goble CA, Bhagat J, Aleksejevs S, et al. myExperiment: a repository and social network for the sharing of bioinformatics workflows. Nucleic Acids Res 38 (2010): 677–82.
- Leinonen R, Sugawara H, Shumway M. The sequence read archive. Nucleic Acids Res 39 (2011): 19-21.
- Langmead B, Langmead Ben. Aligning Short Sequencing Reads with Bowtie. In: Current Protocols in Bioinformatics Inc 5(2010): 11.7.1-11.7.14.
- Dresden, Germany (2022). https://nodepit.com/
Supplementary Material
![]() |
Table S1: Feature consensus Consensus scores are calculated by dividing the number of articles mentioning the criteria by the total number of articles. Features were extracted from the nine manuscripts considered here. The features that we consider critical or important are Indic